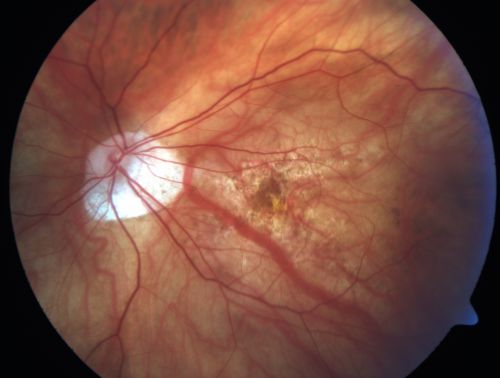
The image above is an optical nerve head plagued with nearsightedness. Image courtesy of Retina Gallery.
Medical imaging of the optic nerve head (ONH) is immensely important to study vision disorders like nearsightedness and glaucoma. Doctors traditionally use optical coherence tomography (OCT scans) to classify optical tissue for study. ONH tissue must be classified in sections, which can become very invasive for patients and sometimes requires testing on animal models.
New opportunities arise from combining advanced imaging like Jones matrix optical coherence tomography (JM-OCT) with machine learning algorithms. The Jones matrix refers to part of a technique to measure a coherent light’s polarization. The vector of incident light is multiplied with the optical element’s Jones matrix to find its polarization. In short, using this polarization study on OCTs reveals more than the OCT alone.
Researchers from the Computational Optics Group at University of Tsukuba, Japan developed a machine learning classification tool based on JM-OCT. They apply this framework to in-vivo sectioning of the optic nerve. In effect, they use k-means clustering to produce criteria for tissue classification. This is a technique where users divide a data set based on each observation’s respective distance from the average. In this case, the OCT image’s pixels are the observations to be clustered. From there, researchers use these pixel clusters to develop their tissue classifications.
Their test case for this classifying method is on ONH subjects that are myopic (nearsighted) or emmetropic (no defects). Cases like these often require continuous OCT and checkups and can fall behind if the patient misreports their symptoms. The researchers aim is to make diagnosing these issues less cumbersome, time consuming and invasive.
Their research can be found here.
What makes JM-OCT different?
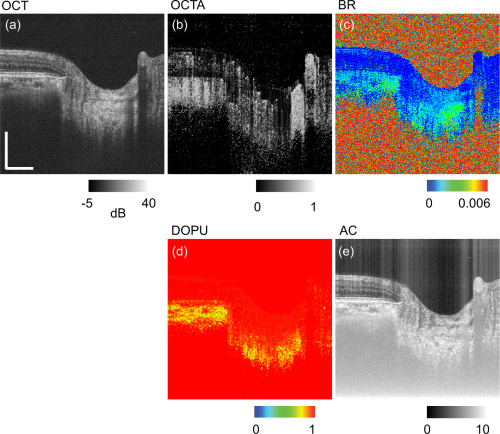
The figure above depicts the contrasts obtained with JM-OCT. Figures a and b are normal OCT, and angiography OCT images respectively. Birefringence is shown in figure c. Figure d shows degree of polarization uniformity (DOPU). Attenuation coefficient is in figure e.
JM-OCT displays more contrasts per single image than normal OCT methods. This technique multiplexes 4 images together: 2 of polarization states and 2 of spectral interferograms. From there, the researchers can cross reference the two sets to look for polarization abnormalities or deviations.
Contrasting these images provides more tools for intricate segmentation and complex tissue analysis. This includes OCT angiography (blood vessel analysis), tissue birefringence and degree of polarization uniformity. Tissue birefringence comes from collagen and may allow JM-OCT to capture the tissue’s biomechanical information.
The researchers use this variety of contrasts as the source for their criteria for tissue classification. They automate the process of cross referencing these images and classifying tissue using this criteria. This enables in-vivo OCT imaging, without the need for numerous OCT scans. Eventually, this process could become more accurate than classification done by a human.
How did researchers create the classification system?
The contrasts produced by JM-OCT are sensitive to different tissue compositions. For example, OCT angiography is sensitive to melanin-containing vascular tissues. The effect is enhanced when contrasted with DOPU and attenuation coefficient. As stated before, birefringence contrasts can detect fibrotic tissues, with lower melanin density in nerve tissues and collagen.
The researchers then remove redundant contrasts from the training database to speed the process up. Certain contrasts, like birefringence x DOPU correlated with simple birefringence analysis. They discarded this contrast and three others with similar correlations.
From here, the researchers performed k-means clustering on a pixel-wise basis. Effectively, the machine automatically matches pixels of each contrast with likewise pixels that indicate similar tissue properties. The algorithm then collects these clusters and lays them over the original OCT scan. This removal of manual segmenting speeds up the automated process, since it has a simple reference case.
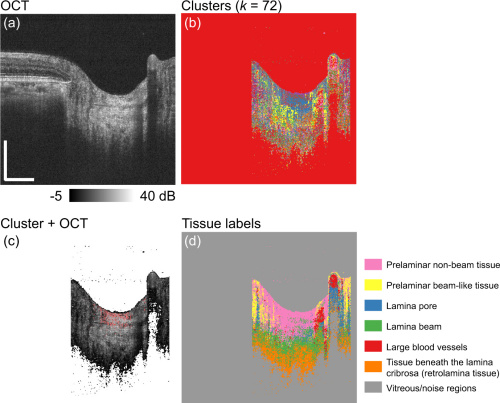
Figures a and b above show the original OCT and the k-means cluster separated. Figure c is of the two overlaid and figure d has color coded clusters for each different tissue that is segmented.
Since the nerve tissues automatically cluster, this already knocks down time constraints that plague manual delineation. The only part of this process that requires direct supervision is teaching the classification database to a machine directly. Manually segmenting the ONH in a patient requires multiple invasive OCT scans and checkups.
Classification System’s Test Results:
As displayed in the previous figure, the dataset clustered the pixels into 7 different tissue categories. Lamina beam tissues are most affected most by myopia, so they were the main focus of the research. Researchers segmented the lamina beam further with Birefringence and attenuation coefficient studies to analyze its biomechanical properties.
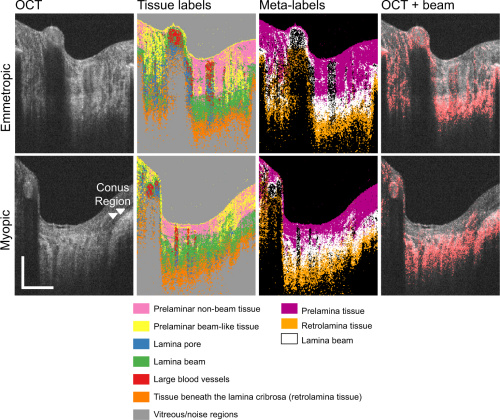
The top row of images are the tissue classification results for an emmetropic case. The bottom row is of a myopic case. Even the untrained eye can see irregularities in tissue distribution in the myopic case. The image labeled OCT + beam is a scan of lamina beam tissue, which is an indicator for myopic and emmetropic conditions.
When compared to manual segmentation, the automated method showed improvements in delineating areas of transition between tissues. Locations of lamina pore and lamina beam regions showed correlated results between both automated and manual segmentation. However, prelamina and retrolamina had much lower correlation because of inherent differences in defining the lamina beam.
Manual delineation can locate lamina pore tissue in correspondence with lamina beams. However, these methods are unable to classify pore tissue as prelamina or retrolamina. Boundaries between certain tissues are simply not sharp or well defined which lead to manual errors.
Overall, the test results displayed high correlation with the automatically generated training database. Areas with large blood vessels are prone to mislabeling and need careful interpretation. A future embodiment of the algorithm may include morphological data to solve misclassification in troublesome areas. However, the automatic generation of a training database is successful as it removes many manual errors in tissue classification.
The data processing field is advancing quickly with new, inventive uses of things like k-means clustering. The researchers left the method open to change from new techniques in segmentation and machine learning.
Conclusion:
The researchers have used machine learning algorithms as an effective step forward in automating the segmentation process. Human error plagues the diagnostic process for these conditions, on both the doctor’s and patient’s end.
Patients are hesitant to seek care for these conditions, for many good reasons. First, patient’s risk misjudging the severity of their symptoms, especially in early stages. Going for a checkup can also be a large commitment considering coverage and cost issues. If a problem is found, it usually requires an enormous amount of time and money as well as repeated visits to the doctor, OCT specialists and pharmacies.
The researcher’s use of JM-OCT enables single shot collection of all contrasts needed. The contrasts collected from JM-OCT alone facilitate all testing needs, since there is an existing classification framework. This means a faster and more accurate diagnosis, with subsequently less patient visits and OCT scans leading to an overall decrease in the cost of optical care.