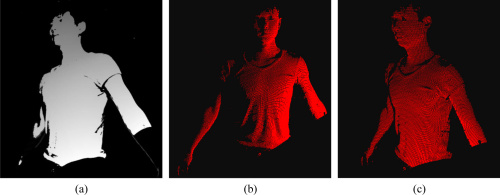
3D body scanning is extremely useful in many fields including healthcare and animation. Courtesy of OSA Publishing.
3D body scanning lends itself to a wide variety of fields. Healthcare professionals can use body scans to make 3D printed prosthetics, animation studios can use body scans for highly-accurate portraits, and apparel companies utilize the scans for custom tailoring. There is no standardized protocol for body scanning as the technology is relatively new. The earliest 3D scanners developed in the 1980s used contact probes placed all over the subject of interest to obtain data points for a digital image. Today, researchers turn to optics for reconstruction.
A research team at Beihang University in Beijing outlines their proposed improvements to 3D body scanning in the October issue of Optics Express. Their approach integrates multiple error-corrective algorithms to optimize scans using structured light technology.
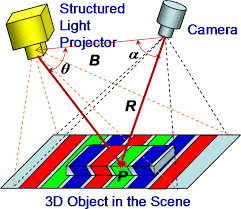
Structured light technology uses a projected pattern to decode information about an object. Courtesy of OSA Publishing.
Structured Light Technology
A simplified structured light technology approach involves projecting one or more patterns onto an object of interest and then capturing the resulting shifted pattern with a camera. We then use the triangulation method to calculate 3D points from this image. Standard algorithms for this process use phase estimation on every pixel to achieve high frame rates.
This becomes more complex when we wish to obtain a full scan from all views, as we need for a 3D image. There are three common strategies to achieve a complete scan: fixing the object while moving the sensor, fixing the sensor while rotating the object, or using multiple sensors on a fixed object. Full body scans currently produce “registration errors” regardless of scanning strategy. These measurement errors result from points reconstructed from different views; errors are especially prevalent between first and last data frames.
The Beihang team set out to lessen these error concerns. Their proposal uses structured light technology with the addition of improved algorithms to better match pixels in camera planes and minimize registration errors.
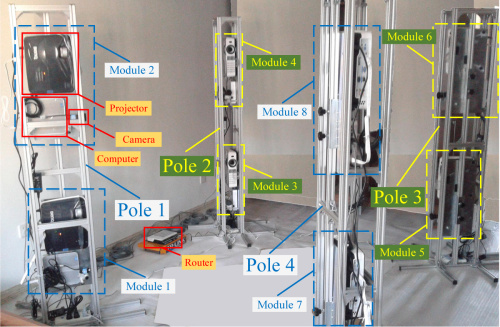
Configuration of the 3D body scanning system. Courtesy of OSA Publishing.
The Setup
The body scanning configuration consists of four poles, each at corners of a square. Each pole carries two modules consisting of a projector, a camera, and a computer. The computer on each module directs the projector to cast fringe patterns on to the body; the camera, in turn, captures the reflection images. Each module aims at the center of the square so that different parts of the body can be scanned.
Phase Shifting and Reconstruction
Structured light-based profiling often requires a four-step phase-shifting algorithm. This method projects four grayscale images with fringe patterns onto the object. The patterns are then altered by the surface of the object and the reflected image registers in the camera. We can then use this information to calculate the intensity of each reflection image on each pixel. The Beihang team’s strategy then takes the extra step of matching pixels in each camera to projector planes using Gray-code method.
A 3D image is ready for construction following some calibration between and within modules. This final step (which is meticulously detailed in the paper) calls for complex analysis of the projection on the image plane, the coordinate matrices for the “world frame,” and the coordinate matrices for the camera. Here, we form the image by determining the coordinates in space that corresponds to each point in the camera.
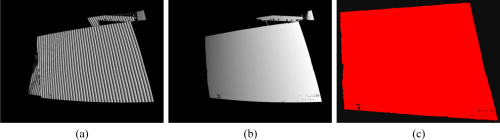
The board across various phases of optimizing. Courtesy of OSA Publishing.
Experiments
Each module produces a 3D point cloud that combine to create the final image.
The team first tested the accuracy of their reconstruction algorithm by imaging a flat board from a single module. Above we see comparisons of optimization steps. Figure (a) shows the relative phase map first obtained by four-step phase shifting, figure (b) is the absolute phase map after applying gray-code, and figure (c) is the final 3D point cloud of the board.
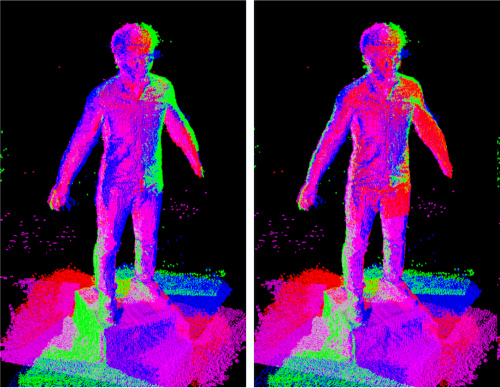
The color distribution allows researchers to see overlap between modules. Courtesy of OSA Publishing.
We can obtain the entire 3D body by reconstructing different parts from each module. Points reconstructed by different modules are represented by different colors. The distribution of colors allows to identify each module’s point cloud so that a graph optimization algorithm can better overlap them for a more cohesive image.
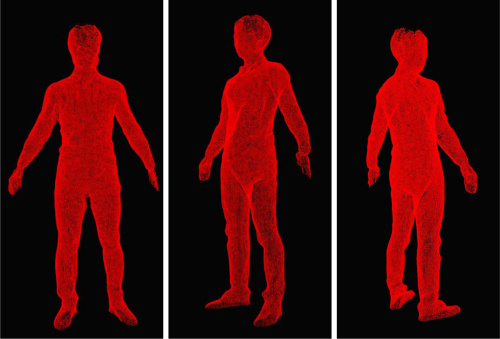
Different views of the final model. Courtesy of OSA Publishing.
Moving Forward with 3D Body Scanning
Multiple optimizing algorithms improve this method of 3D body scanning. These algorithms align point clouds, optimize graphing, and minimize cloud registration errors. Their experimental results show that these algorithms are accurate and allow for complete generation of a 3D human body model. Their method is convenient due to the relatively easy setup. One is able to obtain the complete view without moving the human model or the sensors. Due to their simplistic approach, the Beihang team’s method could soon make a huge impact on healthcare and industry practices.