Introduction
Nowadays, we see the application of artificial intelligence in many places, whether we are a student in a university or an engineer working in an industrial company. Artificial intelligence makes it easier for us to do many things, for example, edit photos and videos of our birthday party, diagnose diseases in hospitals, or even advertise on the internet. So, one would likely find the application of artificial intelligence in the laser industry. Here, in this blog article, we discuss one of the applications of artificial intelligence, specifically, for laser-based additive manufacturing.
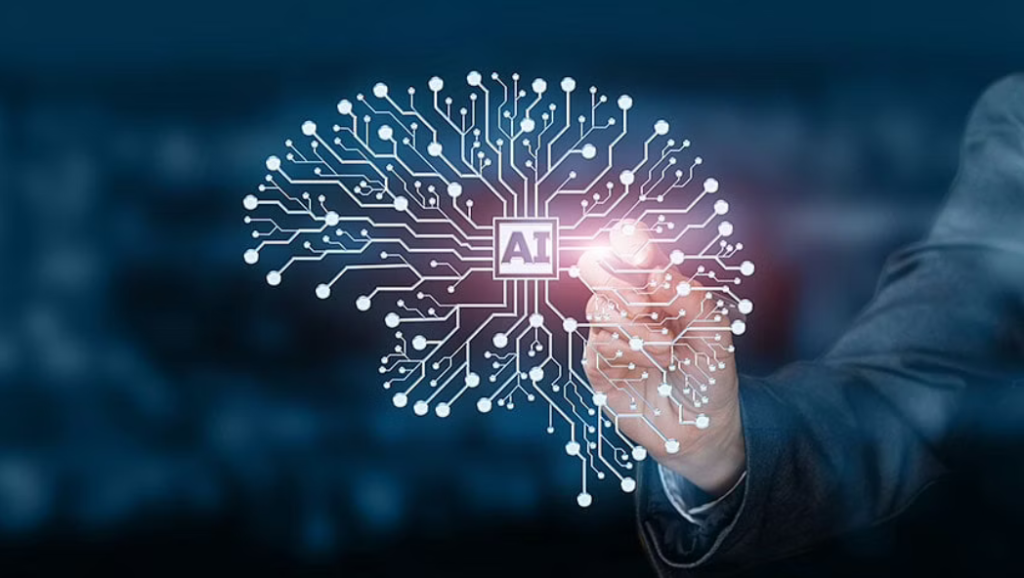
Figure 1: Artificial intelligence (AI) can be seen everywhere nowadays. Photo courtesy of simplilearn
First of all, let’s see what the main challenge with the laser-based additive manufacturing is. In simple words, we can say that Laser-Based Additive Manufacturing (LBAM) is a crucial component of Industry 4.0, which seeks to use numerous sensors for continuous process control. But, what is the challenge? The geometric precision of pieces that are manufactured is a problem with LBAM. Thus, by improving the accuracy of distortion predictions, we can improve the geometric precision.
This is the area where artificial intelligence can help us. Researchers at Mississippi State University have introduced a new artificial intelligence technique that reliably predicts distortion well within LBAM tolerance limits. Specifically, they use “deep learning” based artificial intelligence. Local heat transfer is a key idea in their new technology. Their deep learning technique fits within the Industry 4.0 context of big data analysis with numerous sensors. Now, if you wish to know more about their work, stay with us until the end of this article.
How does thermal history help predict the distortion?
As we discussed in the previous section, we need to increase the geometric accuracy for LBAM such that it becomes a practical choice for industrial manufacturing of mission-critical components. The buildup of residual stress during fabrication is a major cause of geometric error. Repeated heating and cooling during fabrication causes residual stress to accumulate, which ultimately causes distortion of the entire part of interest.
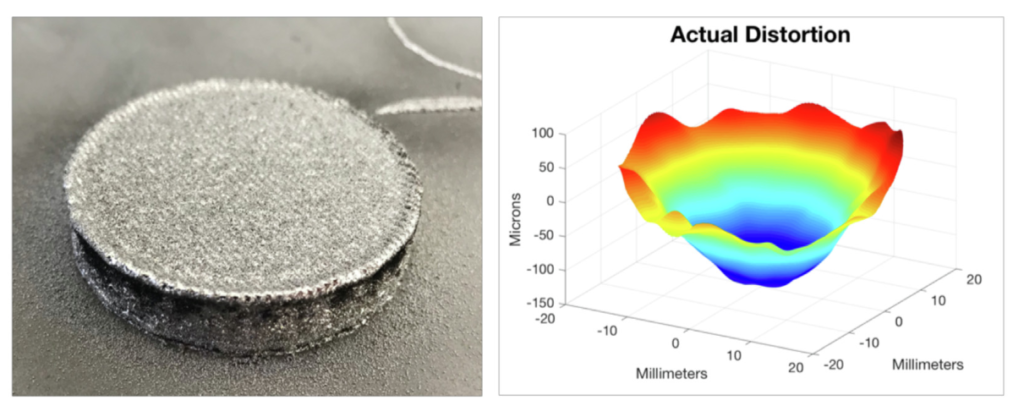
Figure 2: The disk fabricated for the case study and the distortion of the disk; Photo courtesy of Elsevier
Now, if we use the local thermal history, we will be able to anticipate distortion for a variety of geometries. But how?! In simple words, the distortion at each position of the part can be predicted by looking at the heat transfer nearby, in particular, since distortion is generated by the temperature gradient nearby. For example, let’s consider Figure 2. We observe that only the temperature field close to and below the region (shown in Figure 2) significantly affects the distortion. Hence, it is possible to estimate the thermal information (i.e. thermal history) impacting distortion at a given point.
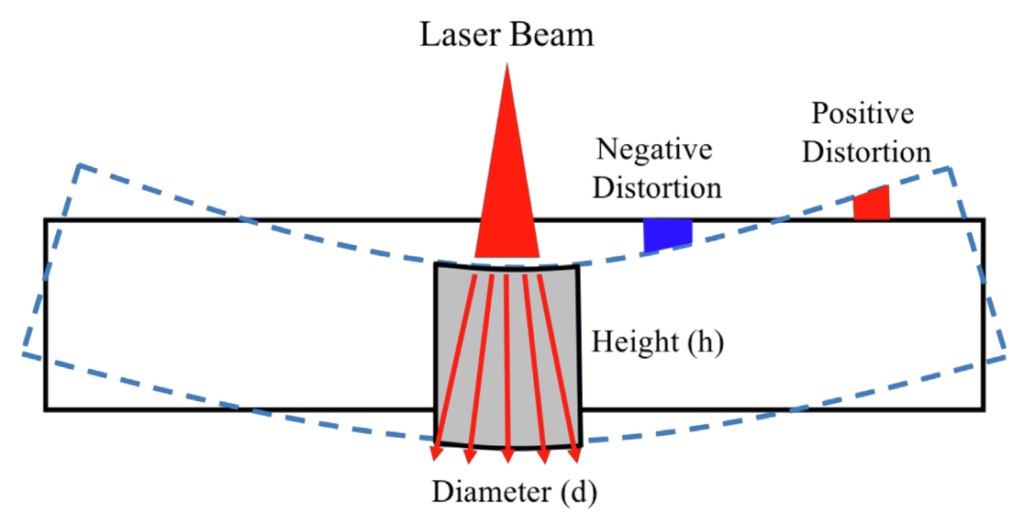
Figure 3: Visualizing the side view of the part during fabrication; Photo courtesy of Elsevier
Employment of artificial intelligent
The researchers create a deep learning model (as a branch of artificial intelligence) that uses thermal history (for example using thermal imaging) to anticipate pointwise distortion. More specifically, they use Convolutional Neural Networks (CNNs) to analyze thermal images as well as a simple Artificial Neural Network (ANN) to include design parameters in the developed artificial intelligence framework. In this framework, the outputs of the CNN and the ANN are combined by concatenation before further training to produce a final pointwise distortion prediction.
The main advantages of this framework are dual in practice. First, by using the deep learning technology combined with high performance computing, we are able to leverage the vast amounts of data collected in the Industry 4.0 context. Moreover, scalability and superior predictive analysis with larger data also become possible. Second, this novel model may be generalized to address other LBAM process control issues including porosity and residual stress.
As a case study, let’s consider the disk shown in Figure 3 along with its actual distortion. Now, we would like to investigate the performance of this novel methodology. Can this methodology really predict the distortion of this disk or not?! If you are excited about the answer to this question, follow us in the next section.
Results of artificial intelligence for a case study
The disk in Figure 2 has a 45 mm diameter and is 5 mm thick. The top surface’s point cloud (167,168 distortions) is created to depict the disk’s pointwise distortion (Figure 3). During the fabrication process, thermal images are recorded using a coaxial, dual-wavelength pyrometer. The research team records a total of 21,818 thermal pictures, creating 40 GB of thermal data. By comparing the distortion at each point on the disk surface to the surrounding thermal pictures and process/design factors, the deep learning framework is trained. They use the supercomputing cluster at Mississippi State to train the deep learning framework. The framework requires a huge amount of memory; therefore training takes 26 days (260 epochs) and 512 GB of RAM.
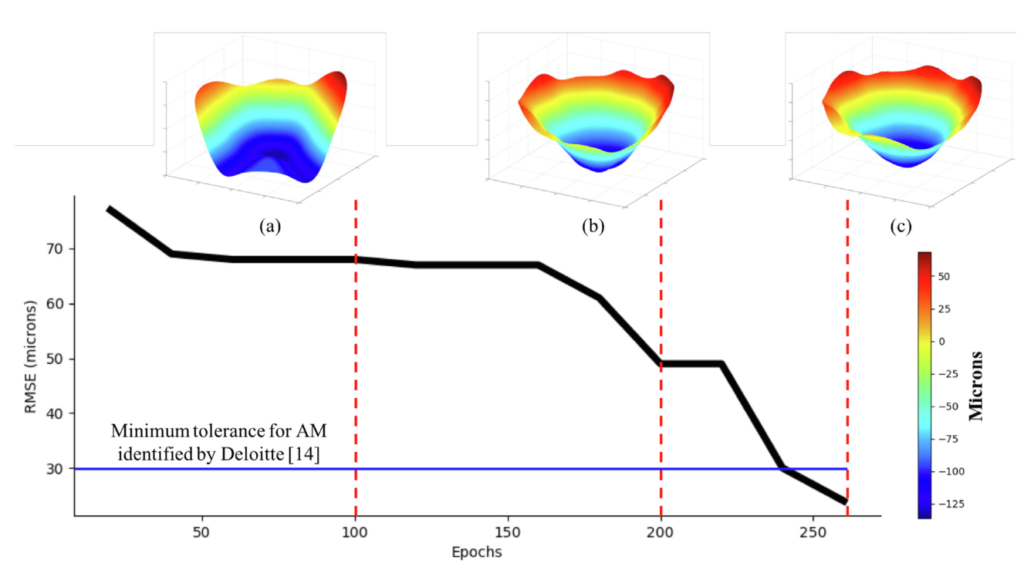
Figure 4: Distortion prediction for the additively manufactured disk; Photo courtesy of Elsevier
Figure 4 displays the outcomes of the actual distortion and the expected distortion. As we can see in this figure, the error of prediction by the deep learning framework decreases by increasing the number of iterations (i.e., epochs). More interestingly, we can observe that the prediction error satisfies the criterion of minimum tolerance, showing a promising result. This outcome demonstrates that the distortion for a disk in LBAM can be precisely predicted by combining thermal history and location.
Conclusions
At the end, let’s summarize and quickly review what we presented in this blog article. In laser-based additive manufacturing, we must improve the geometrical accuracy of fabrication in order to speed up the adoption of Industry 4.0. The novel methodology that we discussed in this article provides four key advantages. First, it cleverly uses “big data” and makes a connection between cloud-computing and manufacturing environment of Industry 4.0. Second, it is based on a deep learning algorithm as an efficient branch of artificial intelligence. Third, its predictions use thermal data together with process and design characteristics. Finally, this novel technology is suitable for various domains with complex shapes and complicated geometries.
I hope you enjoyed reading this article and found it useful. In future articles, we will write about more recent applications of artificial intelligence in the field of laser technologies.
This article was brought to you by Gentec-EO - a leading provider of solutions in photonic sensing and laser beam measurements.