Introduction
A number of disciplines, including flow analysis, particle/cell dynamics and monitoring, and microfluidics, have used digital holographic microscopy. Holograms are diffraction patterns that encode three-dimensional (3D) information, which can be used to decode various types of information. In this article, we discuss the recent research conducted by Pohang University of Science and Technology. Field-portable devices were equipped with in-line type holography, and a portable device was made relying on a super-resolution approach that makes use of multiple images. The prospective use of digital holographic technology have increased as a result of these applications. Because the fringes in a hologram are related to the fine details in the image, the quality of reconstructed holograms depends on the image capturing system’s resolving power. Because of this, high magnification microscope objectives have been used to study the dynamics of particles or cells.
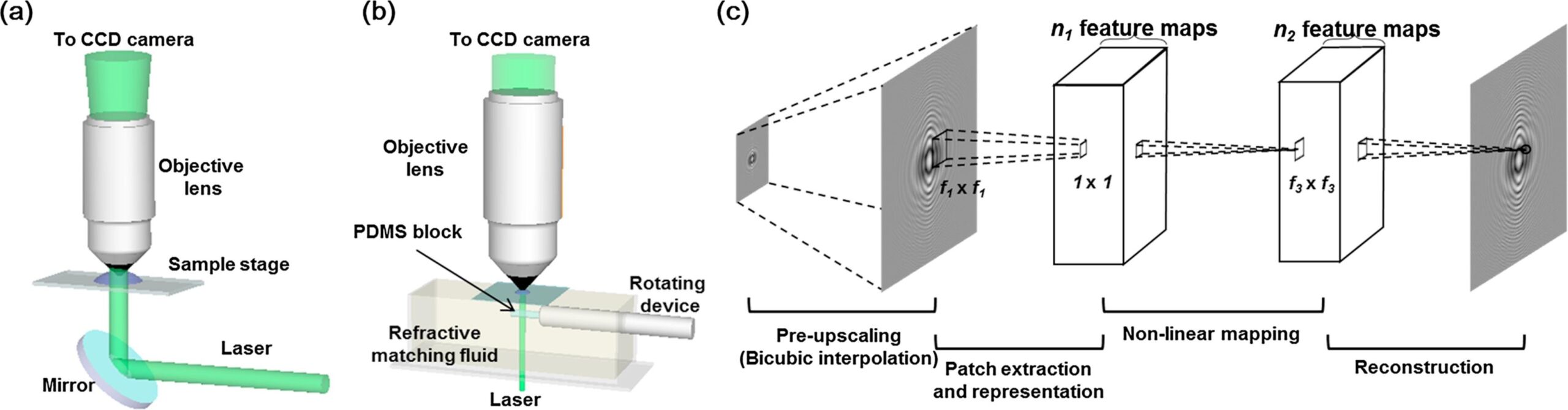
Figure 1: Schematic of digital holographic microscopy (DIHM) setup for recording holograms of moving particles as well as the deep learning procedure. Image courtesy of Elsevier
Artificial Intelligence in Digital Holographic Microscopy
Earlier investigations sacrificed great resolving power by restricting the field-of-view (FOV) to a limited area spanning fewer samples. However, a broad FOV is recommended for the monitoring system when examining the dynamic behavior of particles or cells in flow conditions since these objects frequently travel great distances while exhibiting discrete motions.
Additionally, by increasing the target region, the high FOV can improve particle/cell monitoring effectiveness. Investigating specific details of particle/cell dynamics is challenging due to the trade-off between high resolving power and broad FOV. The applicability and effectiveness of the deep learning technique (see Figure 1) have drawn a lot of attention in a variety of academic fields.
Several neural network techniques were created for a variety of applications when convolutional neural networks (CNNs) were introduced in the imaging disciplines, including image/cell classification, image enhancement, and noise reduction. An example of the associated CNN is shown in Figure 1.
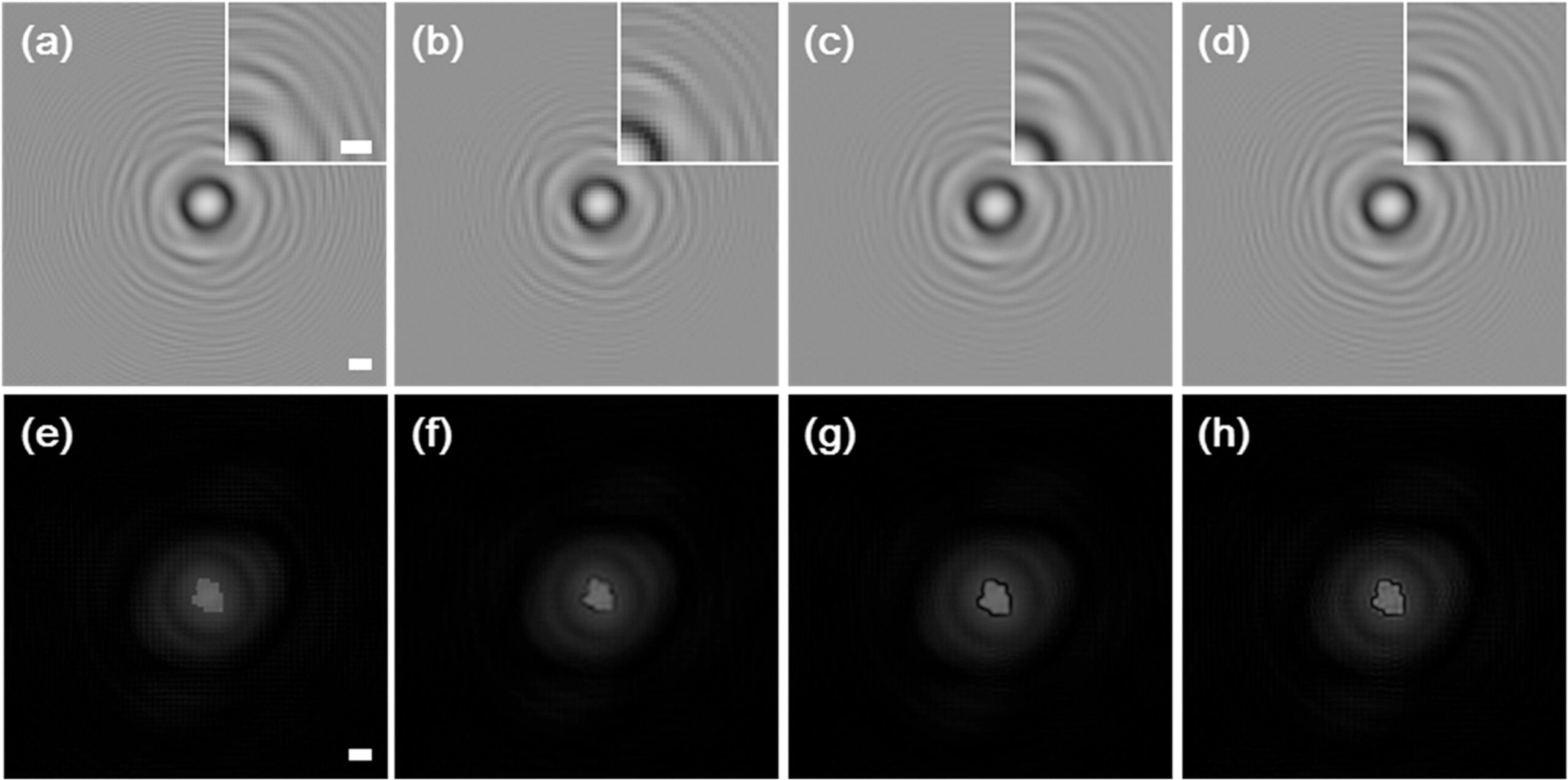
Figure 2: (a) Arbitrary-shaped sample object used for quantitative analysis. (b) High-resolution hologram of the sample object (a). (c) Low-resolution hologram of the sample object. Image courtesy of Elsevier
Challenges with Previous Deep Learning Methods
Many academics have looked at the network for super-resolution (upscaling) images (see Figure 2). A deep learning-based upscaling method has drawn a lot of attention since some researchers proposed a super-resolution convolutional neural network (SRCNN) for a single image super-resolution (SISR). Its performance was enhanced in a number of ways by utilizing new technologies, such as light-field pictures and alternative algorithms with distinct image types.
The deep learning-based SISR method has many technological advantages over earlier upscaling methods that used multiple images. The key benefit is that upscaling only requires one image. The trained network can also be used with different kinds of images. Images of various biological cells can be improved and in-depth information can be gained by using a similar technique of upscaling method on microscope images (see Figure 2).
A deep learning technique was used to carry out the aberration compensation and phase recovery processes in the quantitative phase imaging (QPI) technique. When compared to traditional imaging techniques, these applications concentrated on correcting informational errors from holograms and achieved outstanding results. Prior research, however, mostly dealt with concentrated images with distinct borders and texture.
Even though neural networks for image enhancement, such as SRCNN, can be applied directly to other imaging techniques, their efficacy and use are still unknown. As a typical illustration, for instance, the volumetric recording method of in-line digital holographic imaging technique takes advantage of the diffraction patterns of defocused images (e.g., see Figure 3).
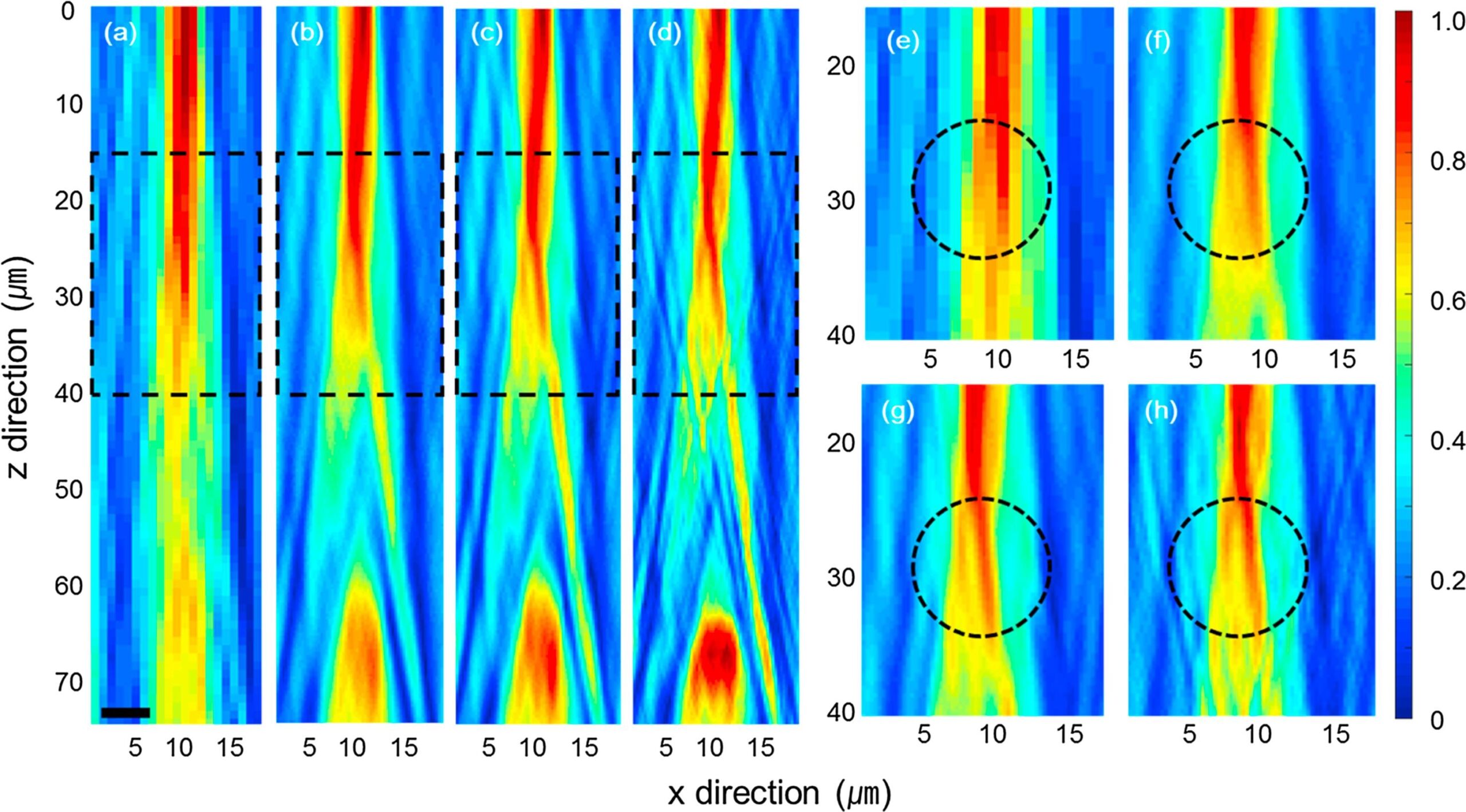
Figure 3: Intensity profiles stacked along the major axis of the prolate particle in the direction of optical axis. Image courtesy of Elsevier
Image Reconstructions in Digital Holographic Microscopy
Phase retrieval, precise localization or autofocusing, and boosting the reconstruction process were some of the areas that were the subject of previous studies combining digital holographic microscopy (DIHM) with deep learning approaches. Additionally, test samples were classified using reconstructed pictures. However, the use of a diffraction pattern to improve the image of a raw hologram is still hypothetical.
The upscaling network trained with focused pictures may in this situation produce unintentional subpar outcomes. As a result, a new hologram trained network is needed, and its utility should be assessed. In this study, DIHM was used with the SRCNN to create high-resolution holograms out of low-resolution ones.
A convolutional neural network that had just been trained on defocused hologram pictures was utilized to create upscaled holograms. By contrasting the upscaled hologram with the high-resolution hologram, the upscaling quality of holograms was confirmed.
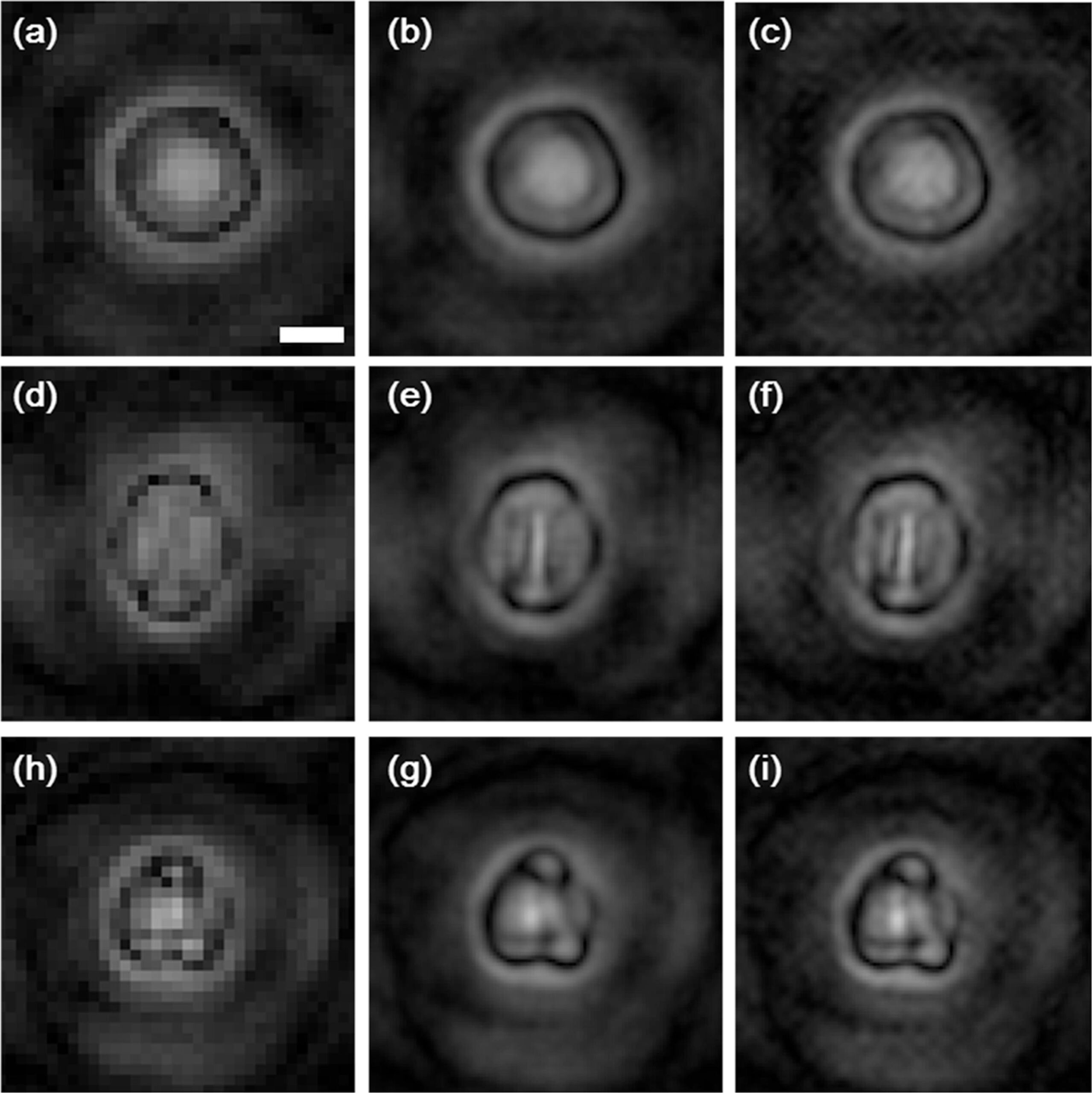
Figure 4: Reconstructed images using a deep learning algorithm. Image courtesy of Elsevier
Comparison to traditional upscaling techniques
In comparison to traditional upscaling techniques, the hologram picture upscaled with deep learning networks offers greater quality in diffraction patterns and reconstruction. It makes it possible to thoroughly analyze the sample object using high-resolution 2D images (e.g., see Figure 4).
Moreover, by examining reconstructed images (see Figure 4) at various reconstruction depths, features in the light scattering pattern might be captured. While this is going on, a low-magnification objective lens can be used to keep the FOV big. In this way, the digital holographic microscopy with deep learning-based upscaling method may resolve the trade-off between the FOV and resolving power, which is important for evaluating particle/cell dynamics and monitoring.
Smaller effective pixel sizes are mostly connected to higher resolving power in image upscaling. The quality of hologram reconstruction is determined, in particular, by the restoration of tiny fringes. The upper right side of holograms made from a spherical particle and recorded with two different microscope objectives is considered.
Important features of high-resolution holograms
In contrast to high-resolution holograms, low-magnification low-resolution holograms do not include high-frequency information. This low-quality hologram can be created by scaling a high-resolution image and matching the pixel size to that of a low-magnification image.
As a result, it may be inferred that the high-resolution image is compressed into the low-resolution image. As a result, decoding a compressed image into an uncompressed one is analogous to restoring a high-resolution image. Small fringes cannot be restored by the bicubic interpolation approach since it only takes surrounding pixels into account.
The hologram reconstruction using HG-SRCNN offers improved quality since the tiny fringes carry detailed high-frequency domain information. Typical deep learning-based upscaling techniques, such SRCNN, concentrate on image edge enhancement and texture restoration.
But since a hologram is essentially a defocused image, improving the fringe pattern is crucial. As a result, as compared to SRCNN combined with holographic microscopy, which is trained with holograms, the standard SRCNN offers comparatively low restoration quality.
Summary and Conclusion
Based on the research conducted by the scientist at Pohang University of Science and Technology, the upscaling method based on deep learning that was created for high resolution and wide FOV was successfully applied to DIHM. Holograms, which are essentially defocused images with diffraction patterns, served as the training data for convolutional neural networks. The trained network was shown to deliver greater image restoration quality compared to the traditional upscaling method using the holograms produced by simulation and experiment. The suggested approach also offers thorough 3D data, including patterns of light scattering. The proposed deep learning-based DIHM approach has two key benefits: monitoring several samples in a wide field of view and assessing particle or cell dynamics using intricate 3D data that was recovered from the upscaled holograms.