Hyperspectral imaging cameras represent a critical advancement in optical sensing, enabling the capture of detailed spectral information across a wide range of wavelengths. Unlike traditional imaging systems that capture only intensity and color information, hyperspectral cameras record a full spectrum at each pixel, making them indispensable in applications requiring precise material identification and analysis. This guide examines the engineering principles, key components, and real-world applications of hyperspectral imaging cameras. It also addresses emerging trends, challenges in adoption, and potential directions for future development, offering a comprehensive resource for scientists, engineers, and professionals leveraging this transformative technology.
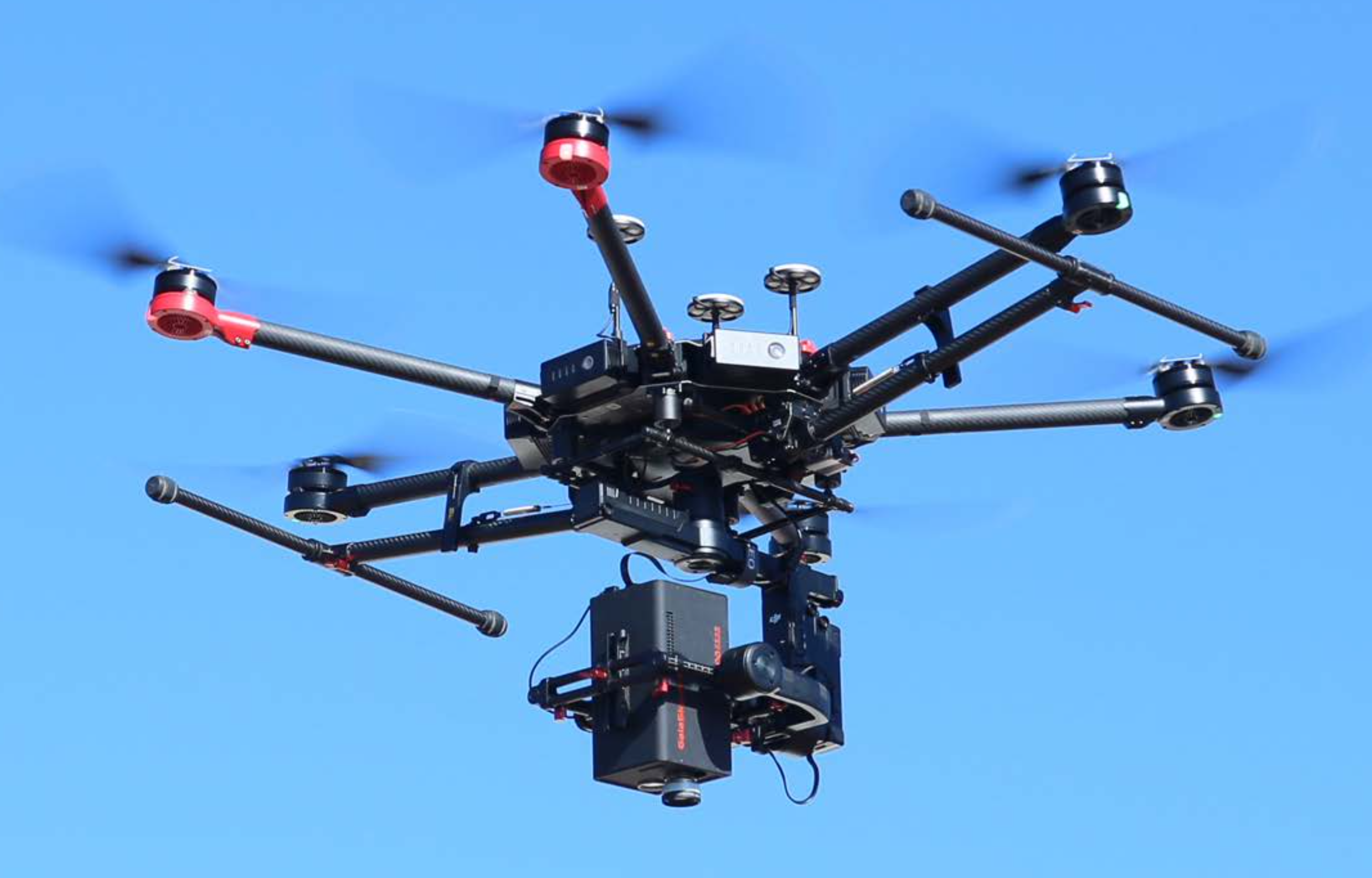
GaiaSky-VIS/NIR Camera: High-resolution hyperspectral imaging achieved through lens scanning and slit spectra projection. Integrated with DJ UAV, it automates data capture across pre-set locations, storing results in an onboard NUC for analysis. Image courtesy of Zolix Instruments. You can find the actual product here: Gaiasky-mini Hyperspectral Imaging Camera
This article is brought to you by Zolix Instruments - Empowering Precision Optics with Innovative Instruments
1. Introduction
Hyperspectral imaging (HSI) is an advanced imaging technique that captures and processes information across a continuous spectrum of wavelengths, often spanning ultraviolet (UV), visible (VIS), near-infrared (NIR), and shortwave infrared (SWIR) regions. Unlike conventional imaging, which captures only three bands (red, green, and blue), HSI records hundreds of narrow spectral bands, creating a detailed spectral signature for every pixel in the image. This spectral data enables precise material identification and differentiation, making HSI invaluable for applications where minute variations in composition or condition are critical.
The origins of HSI trace back to remote sensing efforts in the 1970s and 1980s, particularly in satellite-based Earth observation systems. Early systems, such as NASA’s AVIRIS (Airborne Visible/Infrared Imaging Spectrometer), laid the groundwork for modern HSI technologies by demonstrating the potential of high-spectral-resolution imaging for environmental and geological studies. Over time, advances in sensor technology, computational power, and miniaturization have expanded the use of HSI cameras from large-scale aerial platforms to portable and even drone-integrated systems.
Today, hyperspectral imaging is a multidisciplinary tool with applications across agriculture (crop health monitoring, soil analysis), environmental science (air and water quality assessment), defense (material detection, surveillance), medical diagnostics (tissue analysis, disease detection), and industrial processes (quality control, process optimization). Its ability to reveal unseen spectral details has transformed fields requiring non-invasive, high-resolution material characterization. This guide explores the engineering, data processing, and applications of hyperspectral imaging cameras, offering insights into their current capabilities and future directions.
2. Fundamentals of Hyperspectral Imaging Cameras
Hyperspectral imaging (HSI) operates on the principle of analyzing the electromagnetic spectrum, which spans a wide range of wavelengths from gamma rays (<0.01 nm) to radio waves (>1 m). Hyperspectral cameras typically focus on regions of the spectrum relevant for material analysis, such as the visible (400–700 nm), near-infrared (700–2500 nm), and shortwave infrared (SWIR, 2500–3000 nm) ranges. These regions are particularly valuable for identifying materials based on their unique spectral absorption and reflection characteristics.
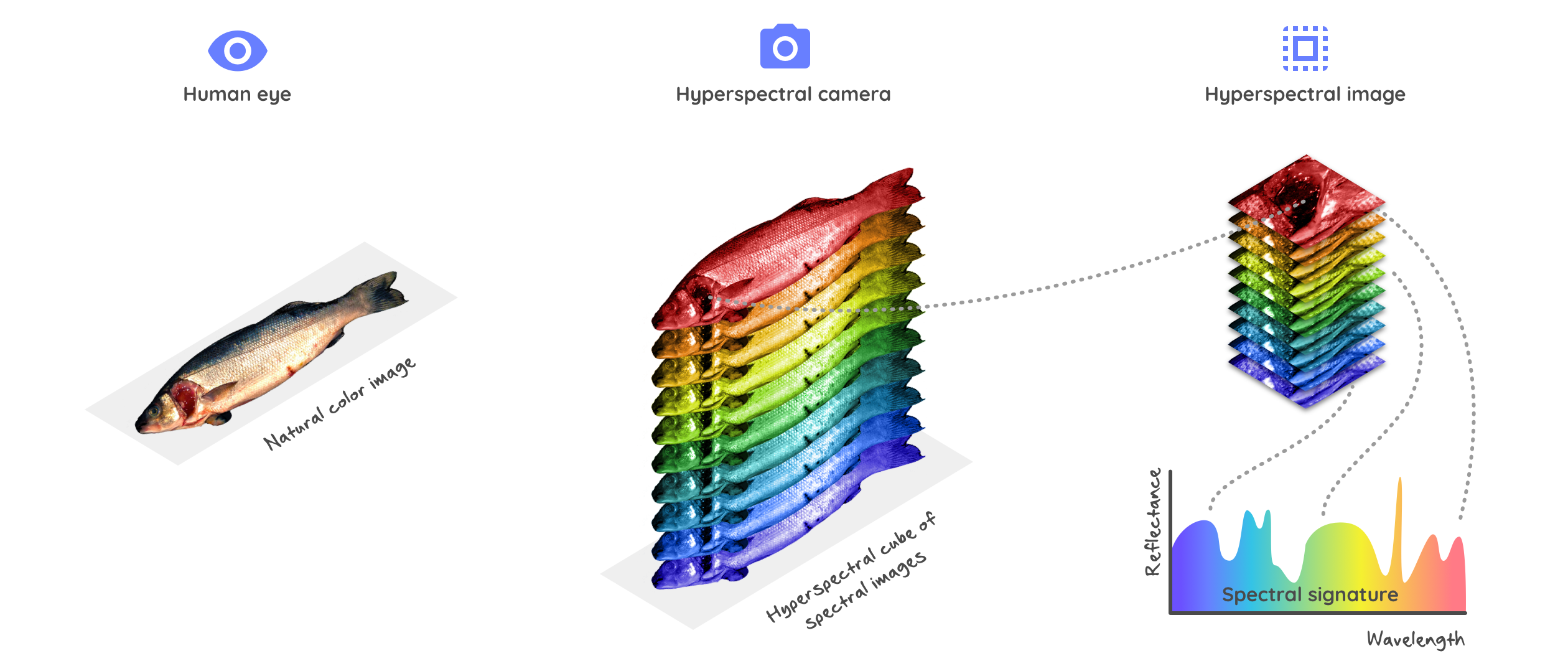
Hyperspectral Imaging Cameras: Hyperspectral imaging differs fundamentally from multispectral and traditional imaging in terms of spectral resolution and data depth. Traditional imaging systems capture information in three broad bands (red, green, blue) to simulate human vision. Multispectral imaging extends this by capturing data in a limited number of discrete spectral bands (e.g., 4 to 12 bands), typically designed for specific applications like vegetation monitoring or mineral mapping. Hyperspectral imaging, however, collects continuous spectral data across hundreds of narrow bands, offering significantly higher spectral resolution. This enables the identification of subtle spectral features, making it possible to distinguish between materials with nearly identical visual appearances. Image courtesy of condi food – Hyperspectral Food Inspection.
Spectral resolution, defined as the narrowness of spectral bands captured by a system, plays a critical role in HSI performance. High spectral resolution allows for detailed characterization of absorption features and is essential for applications like mineral discrimination, water quality assessment, and tissue analysis in medical imaging. However, increased spectral resolution often comes at the cost of reduced spatial resolution and increased data volume, necessitating trade-offs depending on the application.
By providing both spatial and spectral information, HSI creates a “data cube,” where two dimensions represent spatial coordinates (x, y) and the third represents the spectral dimension (λ). This unique capability positions hyperspectral imaging as a powerful tool for material identification, non-destructive testing, and environmental monitoring, among other applications.
3. Engineering Principles of Hyperspectral Cameras
The design and operation of hyperspectral cameras rely on the integration of several key components, each contributing to their ability to capture and process detailed spectral and spatial information. These components must work in harmony to achieve the desired spectral range, resolution, and application-specific requirements.
3.1. Key Components
Sensors and Detectors: Hyperspectral cameras use sensors capable of detecting a wide range of wavelengths, often spanning the visible (400–700 nm), near-infrared (700–2500 nm), and shortwave infrared (SWIR, 2500–3000 nm) regions. The choice of sensor material is critical: silicon-based sensors are common for the visible and near-infrared regions, while indium gallium arsenide (InGaAs) sensors are used for SWIR. These detectors must offer high sensitivity, low noise, and fast response times to accurately capture the subtle spectral features of target materials.
Optics and Spectral Dispersive Elements: Hyperspectral cameras use dispersive optics, such as prisms or diffraction gratings, to separate incoming light into its constituent wavelengths. This dispersion enables the system to collect spectral information for each pixel in the image. For some configurations, filters like acousto-optic tunable filters (AOTFs) or liquid crystal tunable filters (LCTFs) are used to sequentially capture specific wavelengths. These optical components must balance spectral resolution, transmission efficiency, and compactness to meet diverse application requirements.
Data Acquisition and Storage Systems: Hyperspectral imaging generates vast amounts of data, as each pixel contains a complete spectrum. Data acquisition systems must handle high-speed, high-bandwidth signal processing to ensure real-time operation. Storage systems must provide sufficient capacity and write speed to accommodate the large volumes of spectral data, often in gigabytes per dataset. Compression algorithms are frequently employed to manage data without significant loss of spectral fidelity.
3.2. Spectral Range and Resolution Trade-offs
The performance of hyperspectral cameras is defined by their spectral range (the wavelengths they can capture) and spectral resolution (the width of each spectral band). Achieving high spectral resolution typically reduces spatial resolution due to constraints in sensor pixel density and optical design. Similarly, extending the spectral range requires specialized sensors and optical coatings, which can increase system complexity and cost. Engineers must carefully balance these parameters to optimize cameras for specific applications, such as high-resolution tissue analysis or broad-spectrum environmental monitoring.
3.3. Challenges in Miniaturization and Cost Reduction
Miniaturizing hyperspectral cameras presents several engineering challenges. Reducing size and weight often limits optical performance and heat dissipation capabilities, impacting overall efficiency. Innovations in integrated photonics, such as planar waveguides and metasurfaces, are being explored to overcome these limitations. Cost reduction remains another critical focus, as high-performance hyperspectral systems rely on expensive materials (e.g., InGaAs) and precise manufacturing processes. Efforts to develop low-cost, high-performance components, such as quantum dot sensors and mass-producible optics, are key to expanding the accessibility of hyperspectral imaging.
4. Data Processing and Analysis
Data processing is a critical aspect of hyperspectral imaging, converting raw sensor outputs into actionable information. Hyperspectral data are typically represented as a data cube, which includes two spatial dimensions (x, y) and one spectral dimension (λ). This three-dimensional structure provides a detailed spectral signature for every pixel, allowing for comprehensive material analysis.
4.1. Overview of the Data Cube
The hyperspectral data cube represents the spatial distribution of spectral information across a scene. Each spatial coordinate (x, y) corresponds to a pixel, and its associated spectral data (λ) forms a continuous spectrum. This data structure enables detailed differentiation between materials and is the foundation for most hyperspectral analyses. However, the high dimensionality of hyperspectral data poses challenges in storage, computation, and interpretation, necessitating robust data processing pipelines.
4.2. Preprocessing Techniques
Raw hyperspectral data often contain noise and artifacts caused by sensor limitations, environmental conditions, or optical systems. Preprocessing is essential to improve data quality and ensure accurate analysis. Key preprocessing steps include:
- Noise Reduction: Algorithms such as principal component analysis (PCA) or wavelet denoising are used to suppress noise while retaining relevant spectral features.
- Radiometric Calibration: Adjusts sensor responses to account for variations in light intensity, ensuring consistent spectral data.
- Geometric Correction: Aligns hyperspectral images with spatial reference systems, especially for airborne or satellite systems.
- Spectral Normalization: Removes the effects of varying illumination, such as shadowing or sensor inconsistencies, to standardize spectral data.
4.3. Machine Learning and AI Applications
Machine learning (ML) and artificial intelligence (AI) have become indispensable in hyperspectral data analysis due to their ability to handle high-dimensional datasets effectively. Common applications include:
- Classification: Algorithms such as support vector machines (SVMs), random forests, and deep neural networks (DNNs) classify pixels based on their spectral signatures, enabling material identification.
- Anomaly Detection: Unsupervised ML methods, like clustering or autoencoders, identify spectral patterns that deviate from the norm, useful for detecting contaminants or defects.
- Feature Extraction: Dimensionality reduction techniques, such as t-SNE or UMAP, highlight the most informative spectral features for specific tasks, improving computational efficiency.
- Predictive Modeling: AI models can predict material properties, such as chemical composition or stress states, from spectral data.
4.4. Real-Time Processing Challenges
Real-time hyperspectral processing is crucial for applications like drone-based environmental monitoring or industrial quality control but presents several challenges:
- Computational Demand: The high dimensionality of hyperspectral data requires significant processing power, often necessitating specialized hardware such as GPUs or FPGAs.
- Latency: Reducing latency in data acquisition, transmission, and analysis is critical for applications where immediate feedback is required.
- Storage and Bandwidth: Real-time systems must balance the need to store raw data for post-processing with the constraints of onboard memory and communication bandwidth.
- Algorithm Efficiency: Developing lightweight, efficient algorithms that can process hyperspectral data in real time is a major area of research.
By addressing these challenges, the integration of advanced preprocessing techniques and AI-driven analytics continues to enhance the potential of hyperspectral imaging, enabling faster, more accurate, and application-specific insights.
5. Key Applications of Hyperspectral Imaging Cameras
Hyperspectral imaging (HSI) has become a powerful tool across various industries, offering the ability to analyze materials and environments with unparalleled precision. Its unique capacity to capture spatial and spectral data simultaneously makes it indispensable for a range of applications.
5.1. Agriculture
- Crop Health Monitoring: Hyperspectral imaging can detect subtle changes in plant reflectance caused by stress factors such as nutrient deficiencies, pests, or disease. By identifying these issues early, farmers can optimize crop management and improve yield.
- Soil Analysis: HSI provides detailed information on soil composition, including moisture, organic matter, and mineral content. This enables precision agriculture techniques, reducing resource use and maximizing productivity.
5.2. Environmental Monitoring
- Air and Water Quality Assessment: Hyperspectral sensors are highly sensitive to pollutants, allowing for real-time monitoring of air quality and detection of water contaminants like algae blooms, oil spills, or heavy metals.
- Wildlife Conservation: HSI is used to study habitat changes, map biodiversity, and monitor species distribution. It can also detect illegal activities such as deforestation or poaching by identifying spectral signatures unique to vegetation or animal species.
5.3. Defense and Security
- Material Detection and Identification: HSI is widely used in defense for identifying materials, such as explosives or camouflaged targets, based on their unique spectral fingerprints. This capability is essential for threat detection in critical scenarios.
- Surveillance Applications: Hyperspectral imaging enhances surveillance by enabling the identification of concealed objects, distinguishing between similar materials, and detecting anomalies in security-sensitive areas.
5.4. Medical Imaging
- Early Disease Detection: HSI can differentiate between healthy and diseased tissues by detecting subtle spectral changes, aiding in the early diagnosis of conditions like cancer, diabetes-related skin changes, or retinal diseases.
- Tissue Characterization: The technology is used in surgical guidance and research to map tissue composition, oxygenation, and perfusion, offering insights that are beyond the scope of conventional imaging methods.
5.5. Industrial Applications
- Quality Control: HSI is employed in industries such as food production, pharmaceuticals, and manufacturing to identify defects, contaminants, or variations in composition, ensuring product quality and consistency.
- Process Optimization: Hyperspectral systems monitor processes in real time, such as sorting recycled materials or optimizing chemical reactions, to enhance efficiency and reduce waste.
Each of these applications leverages the unique strengths of hyperspectral imaging to address complex challenges, improve decision-making, and enhance operational outcomes across scientific and industrial domains.
6. Innovations and Trends for Hyperspectral Imaging Cameras
Advances in sensor technology are driving significant improvements in hyperspectral imaging. Quantum dot sensors, with their tunable bandgap properties, are enabling more compact and efficient detectors that cover broader spectral ranges. Similarly, innovations in shortwave infrared (SWIR) detectors, such as those based on indium gallium arsenide (InGaAs), are enhancing sensitivity and resolution, particularly for applications requiring precise material characterization.
The integration of hyperspectral cameras with unmanned aerial vehicles (UAVs) and robotics is transforming remote sensing applications. UAV-mounted systems provide high-resolution data over large areas, enabling real-time monitoring for agriculture, environmental conservation, and disaster management. Robotic platforms equipped with hyperspectral imaging are increasingly used in industrial environments for automated inspections and quality control.
Emerging computational imaging techniques, such as compressed sensing and deep learning-based reconstruction, are addressing the challenges of processing large hyperspectral datasets. These methods reduce the computational burden, improve data acquisition speed, and enhance image reconstruction quality, making real-time applications more feasible.
The potential for consumer-grade hyperspectral cameras is becoming a reality as miniaturization and cost-reduction efforts progress. Compact devices designed for specific applications, such as health monitoring or personal environmental assessments, could bring hyperspectral technology to broader markets. These trends suggest that hyperspectral imaging will continue to expand its reach, offering new possibilities across scientific, industrial, and consumer domains.
7. Challenges and Future Directions
Despite their transformative potential, hyperspectral imaging cameras face several technical and practical challenges. One of the primary barriers is the high cost of hyperspectral sensors and associated hardware, which limits widespread adoption in cost-sensitive industries. Additionally, the vast amounts of data generated by hyperspectral systems demand significant computational resources for storage, processing, and analysis, posing challenges for real-time applications and portability.
Another major challenge lies in standardization. The lack of universal protocols for data acquisition, calibration, and analysis hampers cross-comparison and interoperability between systems. This is particularly critical for industries such as healthcare and environmental monitoring, where consistent and reproducible results are essential.
From a technical perspective, improving spectral resolution while maintaining compactness and affordability remains a core focus. Innovations in sensor materials, such as quantum dot detectors and MEMS-based dispersive elements, are promising but require further development to achieve commercial viability.
Future advancements in hyperspectral imaging are likely to be driven by three key areas: miniaturization, integration, and automation. Miniaturized hyperspectral systems that can be deployed on drones or handheld devices will expand applications in remote sensing and field diagnostics. Integration with artificial intelligence (AI) and machine learning (ML) will enhance the interpretation of spectral data, enabling automated anomaly detection, material classification, and predictive analytics. Automation in data collection and processing, particularly through robotics and autonomous systems, will further streamline workflows and reduce operational complexity.
8. Conclusion
Hyperspectral imaging cameras have emerged as indispensable tools in a wide range of scientific, industrial, and environmental applications. By capturing detailed spectral signatures for every pixel, these systems provide unparalleled insights into material properties, enabling precise analysis and decision-making. Over the decades, advancements in sensor technology, data processing algorithms, and system integration have driven the evolution of HSI from specialized research instruments to versatile devices suitable for diverse fields such as agriculture, defense, healthcare, and environmental monitoring.
Despite their growing accessibility, challenges remain, including the high cost of sensors, the complexity of data acquisition and analysis, and the need for standardized protocols across industries. Addressing these challenges requires continued interdisciplinary collaboration, particularly in sensor innovation, computational efficiency, and application-specific optimization.
Looking ahead, trends such as miniaturization, integration with autonomous platforms like drones, and the incorporation of machine learning for real-time data analysis will further expand the capabilities and applications of Hyperspectral imaging cameras. As these technologies advance, hyperspectral imaging cameras are poised to play a critical role in solving complex problems, driving innovation, and uncovering insights that were previously beyond reach.
9. References
These references provide a comprehensive overview of hyperspectral imaging technologies, their applications, and market trends.
- Hyperspectral Imaging Market Analysis | Industry Growth, Size …
Mordor Intelligence. Retrieved from https://www.mordorintelligence.com/industry-reports/hyperspectral-imaging-market - An extensive review of hyperspectral image classification and …
Multimedia Tools and Applications, 2024. Retrieved from https://link.springer.com/article/10.1007/s11042-024-18562-9 - Hyperspectral Imaging Systems Market Size Report, 2030
Grand View Research. Retrieved from https://www.grandviewresearch.com/industry-analysis/hyperspectral-imaging-systems-market - Improving hyperspectral imaging using a lensless camera
APL Photonics, 2023. Retrieved from https://pubs.aip.org/aip/sci/article/2023/26/261102/2900580/Improving-hyperspectral-imaging-using-a-lensless - Active and Low-Cost Hyperspectral Imaging for the Spectral …
Sensors, 2023. Retrieved from https://www.mdpi.com/1424-8220/23/3/1437 - Hyperspectral Imaging Algorithms and Applications: A Review
TechRxiv, 2023. Retrieved from https://www.techrxiv.org/doi/full/10.36227/techrxiv.24743562.v1 - High-Resolution Hyperspectral Video Imaging Using A Hexagonal Camera Array
arXiv preprint, 2024. Retrieved from https://arxiv.org/abs/2407.09038 - Real-time Hyperspectral Imaging in Hardware via Trained Metasurface Encoders
arXiv preprint, 2022. Retrieved from https://arxiv.org/abs/2204.02084 - Hyperspectral Imaging for Clinical Applications
BioChip Journal, 2021. Retrieved from https://link.springer.com/article/10.1007/s13206-021-00041-0 - Hyperspectral Imaging – Market and Technology Forecast to 2030
Research and Markets. Retrieved from https://www.researchandmarkets.com/reports/5557625/hyperspectral-imaging-market-and-technology