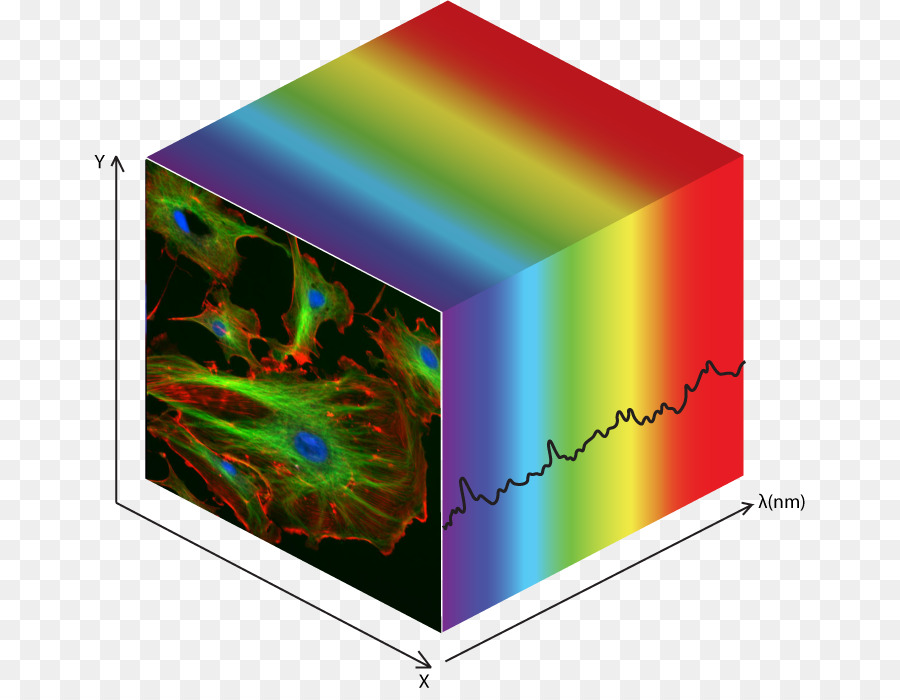
Hyperspectral imaging is an emerging technology for cancerous tumor detection. A research team published their comprehensive study on HSI-based imaging for thyroid and salivary gland tumor detection. Courtesy of CleanPNG.
Thyroid and Salivary Gland Tumor Detection
Salivary gland tumors and thyroid tumors are both considered head and neck cancers, so they are often grouped together when discussing diagnoses or treatments. They may occupy a similar space, but extracting either tumor requires a unique approach. These cancers are located in particularly vulnerable places in the body. Optimizing imaging and detection methods allows surgeons to plan and approach the tumor in the safest way possible.
The American Cancer Society estimates that 53,000 adults in the United States will be diagnosed with thyroid cancer this year. It will be the most common cancer diagnosis in people age 15-29 and men age 30-39. Papillary thyroid carcinoma (PTC) comprises 70% of thyroid cancers. Variants of PTC include conventional, tall-cell, oncocytic, and follicular. Pathologists diagnose thyroid tumors with fine-needle aspiration biopsy and histological evaluation. There is much debate among doctors and researchers as to the accuracy of intraoperative frozen section analysis to determine the cancer’s progression. One study found that frozen sectioning has a 22% sensitivity to identifying malignancy.
Salivary tumors typically involve the major salivary glands including the parotid, submandibular, and sublingual. More than 80% of salivary tumors are found in the parotid, the largest gland. As with thyroid tumors, intraoperative frozen sectioning provides unreliable results: studies find the method operates with a 33% sensitivity.
Introducing Hyperspectral Imaging
Hyperspectral imaging (HSI) utilizes an imaging spectrometer to collect spectral information about the object of interest. The spectrometer (or hyperspectral camera) analyzes thousands of spectra to form an image, and every pixel within the image contains a complete spectrum. Hyperspectral data is therefore three-dimensional, generating a data cube that contains absorption, reflection, or fluorescence spectrum data for each image pixel.
Hyperspectral imaging has proven to be effective in many biomedical applications including both ex-vivo and in-vivo cancer detection studies. A group with the University of Leipzig demonstrated that HSI can differentiate parathyroid glands from thyroid tissue during a thyroidectomy. This method allows the surgeon to leave the parathyroid glads intact during surgery. Similarly, a group with Fraunhofer Heinrich Hertz Institute was able to successfully use HSI to locate the facial nerve in the parotid gland during salivary gland tumor extraction. This greatly reduces the procedure’s risk, as the injuring the facial nerve can cause facial paralysis.
A collaborative team with the University of Texas at Dallas and Emory University seeks to expand their past work with HSI to detect thyroid and salivary gland tumors. Their work published in the March issue of Biomedical Optics Express details the performance of HSI-based tumor detection on 82 total patients.The massive study is the most comprehensive of its kind to date, covering 200 thyroid tissue specimens and 16 salivary gland specimens. They seek to determine the viability of HSI-based imaging for computer-aided tumor detection as well as specific benefits of HSI for thyroid and salivary gland tumor detection.
Ex-Vivo Samples for HSI Tumor Detection
The researchers began by collecting three sample types from each patient: normal tissue, tissue from the tumor, and a sample at the interface between the two. A pathologist then identified and divided the samples to create one group of thyroid tumors and one group of salivary gland tumors. Both cohorts included subgroups. There was five subtypes of malignant thyroid tumors: PTC, MTC, insular carcinoma, follicular carcinoma, and thryroid carcinoma. The pathologist also identified a sixth group of follicular adenoma, benign thyroid tumors. Salivary gland tumor subgroups included the malignant mucoepidermoid carcinoma, salivary duct carcinoma of the parotid gland, PLGA of the hard palate, and adenoid cystic carcinoma. Two patients formed an additional subgroup of benign pleomorphic adenoma of the parotid gland.
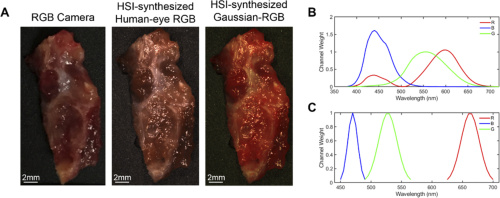
A thyroid tumor sample represented with each multiplex imaging method. Chart B displays the spectral signature of the human eye’s perception of red, green, and blue. Chart C shows the Gaussian kernels that generated the Gaussian RGB images. Courtesy of OSA publishing
Hyperspectral-Based Imaging
The research team compared the performance of HSI-based methods for tumor detection with results from several established optical imaging techniques. These systems included label-free autofluorescence and two fluorescent dyes: 2-NBDG and proflavine.
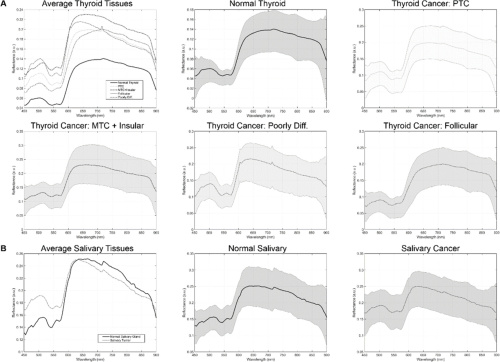
Spectral signatures for the tissue in the thyroid cohort (a) and salivary gland cohort (b). Courtesy of OSA Publishing.
The hyperspectral imaging system uses a Xenon light source and liquid crystal tunable filter to run a spectral scan from 450 to 900 nm. Images of the ex-vivo samples are 1040x1329x91 pixels. These dimensions represent height, width, and spectral bands, respectively. This corresponds to a spatial resolution of 25 microns per pixel. The researchers also calibrated the raw hyperspectral data by subtracting dark current of the sensor and dividing by a white reference disk for each pixel. The above charts show the final spectral signatures from all groups.
The team also generated three types of synthetic composite images from the normalized hyperspectral hypercube. The first three-band RGB multiplex image was formed by applying a Gaussian kernel to each color region of the HSI. The second image was constructed as a reference to human color perception curves, and an RGB camera captured a third multiplex image.
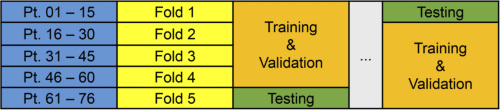
The above schematic depicts the experimental design for the 76 patient thyroid tumor cohort. Courtesy of OSA Publishing.
Deep Learning Methods
This method employed deep learning algorithms built from histological ground truths. Each collected specimen was marked at the top, bottom, left and right edges, and back surface to identify its orientation in histological sections. The researchers imaged 5 micron slices from the surface of each tissue so that a pathologist could annotate the tumor and healthy regions on the digital histology images. A binary mask comprised of these images served as the ground truth for optical images of the specimen.
Deep learning required training, validating, and testing the neural network. The thyroid tumor and salivary gland tumor cohorts were partitioned differently due to their dramatically different sample sizes.
The thyroid cohort consisting of 76 patients produced fully independent inter-patient results. These patients were divided across five folds such that each fold could serve as the independent testing group while the other four folds were trained and validated. The salivary gland cohort of 6 patients produced intra-patient training and testing. The researchers performed training and validation on the patients’ primary tumors and normal tissue; they performed testing on the sample collected at the tumor-normal tissue interface. This cohort split into two groups: 3 patients with tumors of the parotid gland and 3 with other types of salivary gland tumors.
Testing the Neural Networks
The researchers constructed a convolutional neural network (CNN) to classify the 200 thyroid tumor samples as tumor tissue or normal tissue. After training for 23 hours, the CNN could classify a new thyroid tissue sample in an average of 20 seconds for all imaging modalities. Overlapping pixel regions produced heat maps.
The team used a simplified artificial neural network to classify the 16 salivary gland tumor samples with only spectral information. The system extracted pixel block averages to produce spectral signatures with reduced noise. Spectra from normal tissue and tumor tissue contributed to training and validation while spectra from the tumor-normal samples contributed to testing. Training the network took only minutes, and tests ran for about one second.
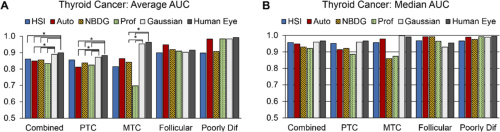
Average and median AUC scores for thyroid tumor detection imaging methods. Courtesy of OSA Publishing.
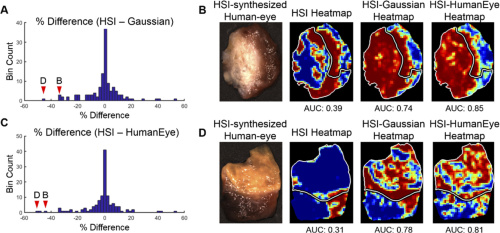
AUC score performance comparing HSI, HSI-synthesized Gaussian-RGB multiplex, and HSI-synthesized human-eye RGB multiplexing. Courtesy of OSA Publishing.
The Results
The researchers evaluated the area under the receiver operator curve (AUC) to determine the performance of all tested imaging modalities. The above table depicts the AUC score, sensitivity, accuracy, and specificity for thyroid tumor detection. Results for thyroid tumor detection demonstrate that HSI-based methods outperformed fluorescent dye-based methods for 71% of cases represented by the PTC group. Notably, HSI-synthesized RGB multiplex images outperformed all other tested imaging methods for all thyroid tumor types. Salivary gland tumor detection results, meanwhile, show HSI was best for parotid glands and autofluorescence performed best for other salivary gland tumor types.
Concluding Thoughts
Results from the thyroid tumor detection study upheld the hypothesis that HSI-based imaging and autofluorescence outperform dye-based imaging methods. The researchers propose that their work indicates many imaging modalities are viable to create deep learning algorithms with a large enough sample size. This presents the potential for future studies with salivary gland tumor detection. Ultimately, the team’s work indicates that hyperspectral imaging methods could greatly enhance conditions for surgeons and pathologists during tumor detection. HSI-based imaging methods have the potential to significantly reduce misdiagnoses rates and minimize risk during tumor extraction.
For more details on multiplexed images, the tested imaging methods, and the generated neural networks, find the team’s paper here.