Laser additive manufacturing and laser powder bed fusion
Recent advances in laser additive manufacturing (AM) have inspired the direct production of functional parts using the technology rather than only fast prototyping. Because of the extensive variety of processing variables, laser additive manufacturing systems are more complicated than conventional manufacturing methods. For instance, the laser power, scan speed, layer thickness, and hatch techniques are all programmable factors in the laser powder bed fusion (L-PBF) process (see Figure 1). Variations in these parameters can produce various microstructure, defect, and thermal behaviors.
Due to the significant impact of microstructure and flaws on fracture initiation, high cycle fatigue is one of the material qualities that is extremely sensitive to processing. The interaction of closely spaced imperfections can speed up fracture propagation and rupture with an increase in defect density.
The consequences of the hierarchical microstructural characteristics, such as directed grains and melt pools at the macro-scale and ultrafine sub-grains, porosities, and inclusions at the micro-scale become relevant as defect size decreases.
The use of the mechanistic fatigue model has not advanced very far due to the variety of L-PBF faults that alter the behavior of the material. Examples include the frequent assumption of small microstructure effects or uniform microstructure layout in defect-based constitutive models. However, it is known that the high temperature gradient in L-PBF processing produces highly heterogeneous and anisotropic microstructures. Characterizing microstructural features, such as grain and inclusion sizes, is difficult due to large part-to-part and build-to-build variabilities and the impact of processing on microstructure formation.
Existing models cannot be used to directly map the links between processes and properties since they do not include L-PBF-related elements. It is associated with higher computing costs and a variety of model sources to tackle the process-structure and structure-property interactions separately using different models.
For example, the process and fatigue life relationship of L-PBF stainless steel 316L can be explored for a two-factor system with the help of a data-driven method that uses the design of experiments technique. Nevertheless, the fatigue failure behavior is too complex to accurately describe using first- or second-order regression equations. Additionally, due to the systematic experimental design preventing the adaptation of data from the literature or the public domain, models created using these methods frequently have a restricted capacity for generalization.
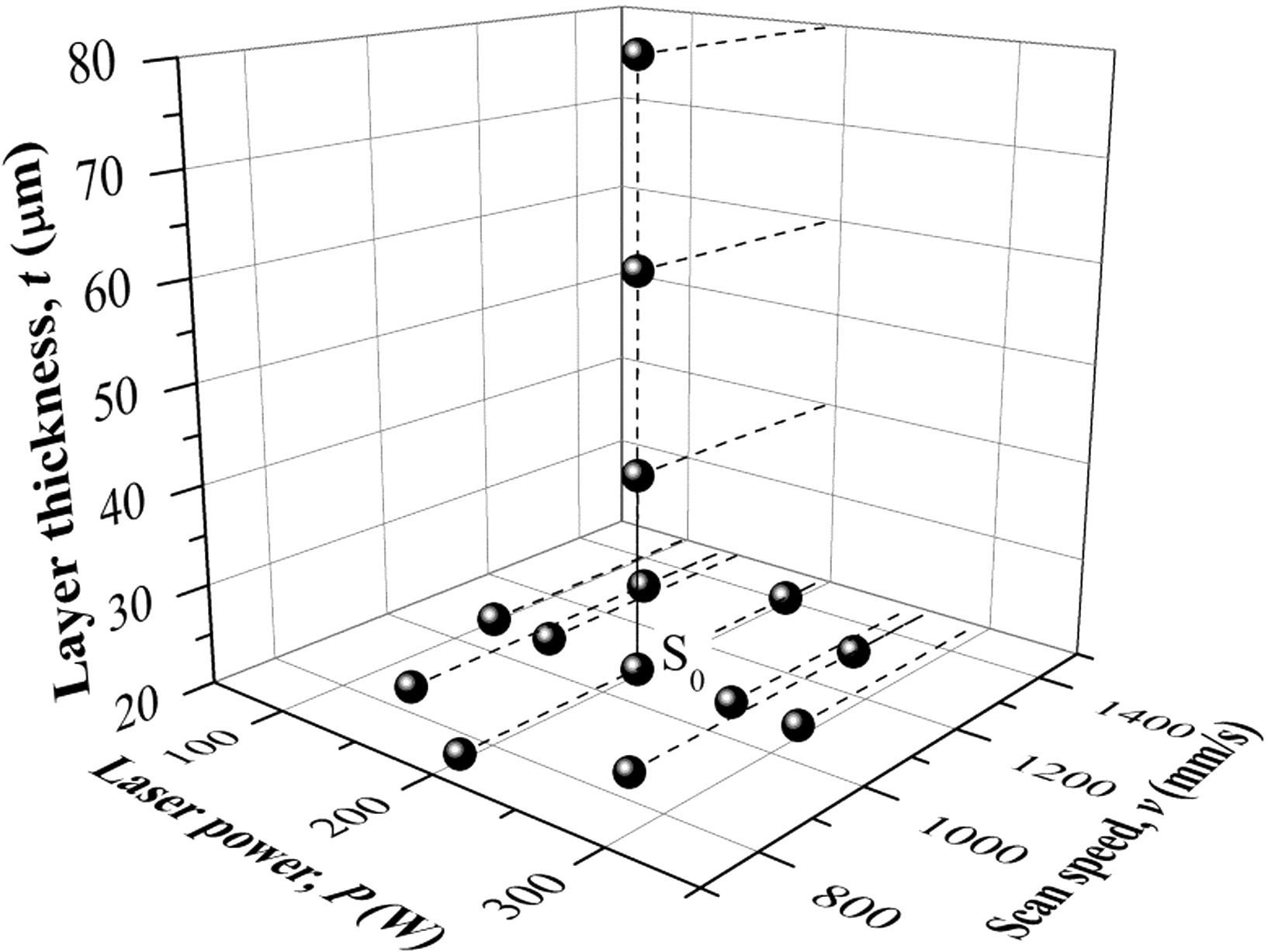
Figure 1: Laser powder bed fusion processing conditions; Image courtesy of Elsevier
In this article, we discuss a research carried out at Nanyang Technological University, Singapore Institute of Manufacturing Technology, and Coventry University. These research groups tested the adaptive neuro fussy inference system (ANFIS), shown in Figure 3, for its ability to predict the high cycle fatigue life of L-PBF stainless steel 316L samples when processing/post-processing circumstances and cyclic stresses were taken into account. Additionally, they used a portion of the dataset, which consists of 139 experimental fatigue data, to train the model. They created two models with the processing/post-processing parameters and the tensile properties as their respective inputs. By using the models to analyze the test data and cross-validating them against findings from the literature, they assessed the performance of the models.
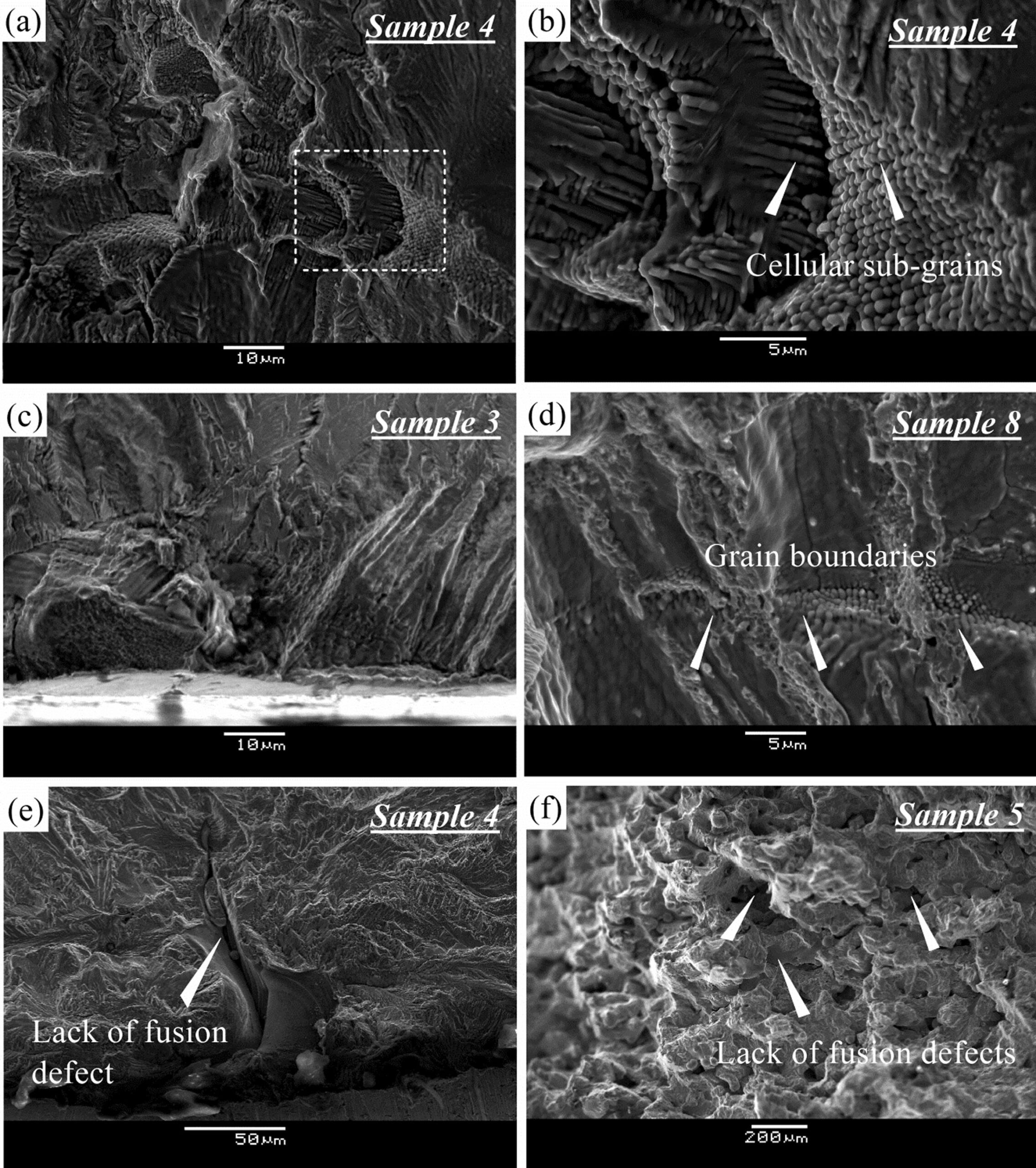
Figure 2: SEM fracture images showing crack initiation; Image courtesy of Elsevier
Data collection for a laser additive manufacturing case
The researchers illustrate how fatigue works probabilistically in L-PBF stainless steel 316L, as an example. When L-PBF was processed, competitive grain growth caused non-equilibrium solidification, which led to the production of second phase and dislocation particles at the grain and sub-grain borders, as well as the formation of differentially oriented grain clusters.
Intergranular cracking was facilitated by the large local stress concentration at the grain boundaries (see Figure 2). At the crack’s beginning, densely packed ultrafine cellular sub-grains are plainly visible, indicating intergranular cracking.
The switch from intergranular– to transgranular-dominated fracture occurred at greater energy inputs in this processing region, as shown in Figure 2c for Sample 3 and Figure 2d for Sample 8, where cracking is particularly sensitive to microstructural heterogeneities.
The transgranular fracture may have resulted from the second phase particles preferentially clustering at the sub-grain boundaries rather than the grain boundaries due to the longer diffusion period at a slower cooling rate. A further increase in energy input led to overheating, where the degrading of material properties was brought on by the evaporation of alloying elements.
Large irregular absence of fusion flaws were created with a decrease in the laser energy input due to inadequate layer-layer and track-track overlaps. Defect-driven crack initiation was caused by intense stress fields along the sharp edges of the flaws, as depicted in Figure 2e. The faults grew bigger and more numerous when the energy input was further reduced. As a result of the interactions between the closely spaced flaws, which amplified the stress fields, many defects simultaneously started to crack, as illustrated in Figure 2f.
Note that crack initiations for Sample 4 were seen to be caused by both microstructure-driven (Figure 2a) and defect-driven (Figure 2e) factors. Both failure modes may be possible depending on the microstructure and defect arrangements, such as size, orientation, and location, as the defects produced at this processing condition may be getting close to the critical size that causes the switch from microstructure-driven to defect-driven crack initiation.
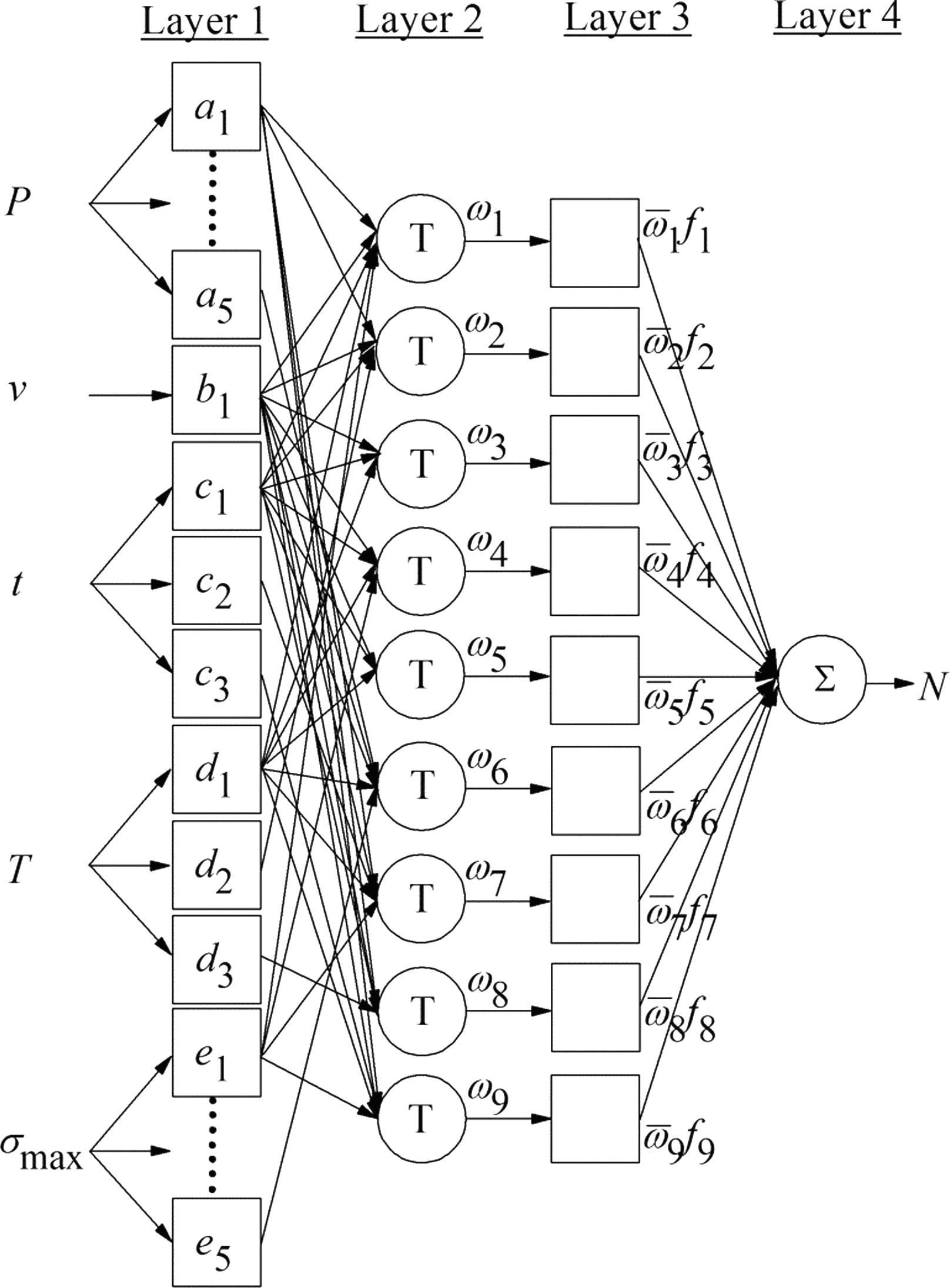
Figure 3: Structure of the adaptive neuro-fuzzy inference system (ANFIS); Image courtesy of Elsevier
Some results and a few take-home messages
The high cycle fatigue life of L-PBF stainless steel 316L was modeled in the work by the researchers using the adaptive neuro-fuzzy-based machine learning technique (Figure 3). The results obtained could lead to the following conclusions:
- The L-PBF stainless steel 316L samples with a wide range of material characteristics and fracture behaviors, resulting from the usage of various processing/post-processing conditions, and subjected to various cycle stress levels, were correctly predicted by the ANFIS technique to have a fatigue life (see Figure 4 for a few prediction results). The models successfully captured the common failure modes in the dataset, which served as the foundation for the rules used to forecast fatigue life.
- The model could be appreciated more thanks to the transparency provided by the language norms, which also made it easier to choose model parameters and streamline the design and validation processes. The ANFIS has this benefit over non-fuzzy-based learning strategies. Additionally, using fuzzy boundaries might have allowed for improved data tolerance, allowing the models to more effectively account for the scatter in the S-N data.
- The capacity of the model to generalize is improved by choosing representative input variables and building a sizable database for training.
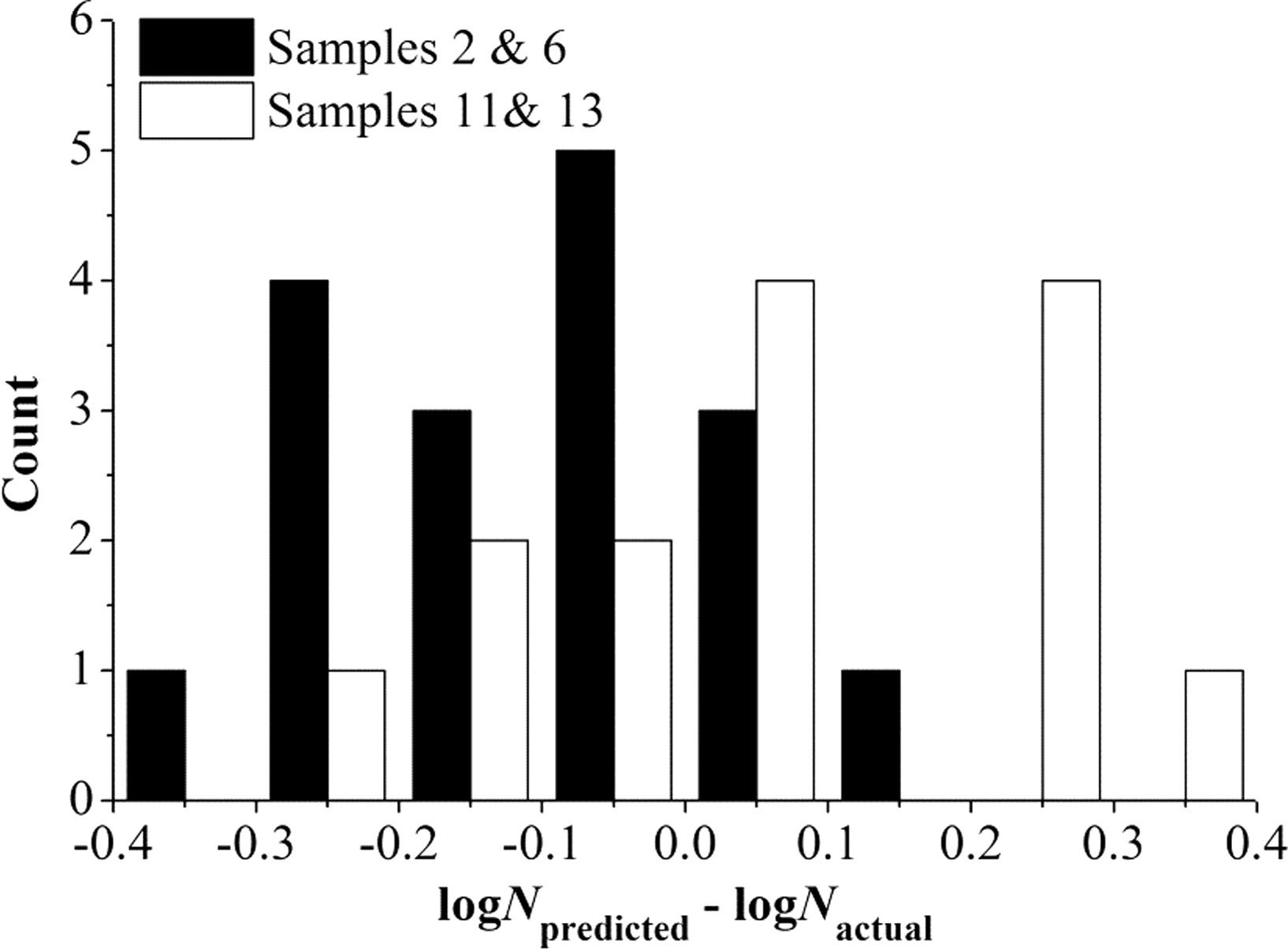
Figure 4: Histograms showing the error distributions for a few samples; Image courtesy of Elsevier