Introduction to laser-aided additive manufacturing
We usually use a powerful laser beam in laser-aided additive manufacturing (LAAM), also known as Laser Metal Deposition (LMD) or Directed Energy Deposition (DED), to melt wire or blown powder jet feedstock for metallic additive manufacturing. By using the concept of increasing the amount of material to construct a component, as opposed to more traditional material reduction technologies like milling and turning, LAAM improves building efficiency while lowering design constraints and material waste. These characteristics encourage the widespread application of LAAM technology in the creation of 3D (three-dimensional) parts.
The laser beam movement tactics or toolpaths are referred to as laser scanning patterns in LAAM. The temperature distribution over the entire component will be significantly impacted by the deposition paths, particularly for multi-layer deposition processes, leading to unique residual stress distributions and substrate deformations.
Due to variations in temperature distribution and cooling rates, the part microstructure and mechanical characteristics will also be different. Therefore, choosing the right laser scanning pattern is essential for reducing overall distortion and improving the component’s structural qualities. Due to our incomplete understanding of the complicated multi-physics phenomena underlying laser-aided additive manufacturing, current laser scanning selection primarily depends on empirical experience.
An overview of laser scanning pattern strategy
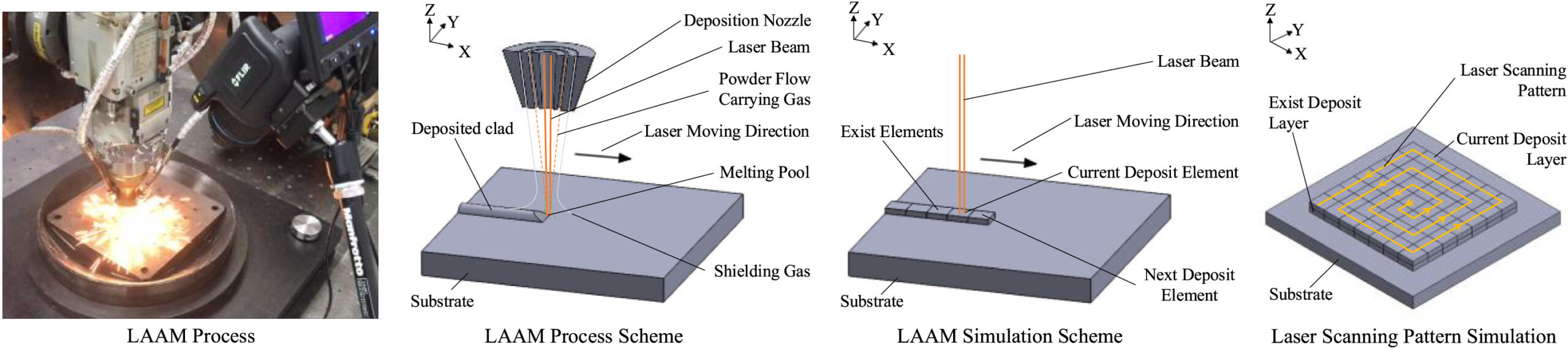
Figure1: The scheme of finite element model evolution; Photo courtesy of Elsevier
The primary goals of the laser scanning pattern strategy (such as “optimizing method“, “medical axis” method, and “sub-region generation” method) are to identify the material deposition paths without collision and to completely cover the 2D cross section profile in each layer. The scanning strategies’ impact on the temperature distribution, as well as their impact on the distribution of residual stresses and substrate deformation, are not taken into account by these methods.
To investigate the relationships between various process parameters, thermal behavior during deposition, and the mechanical properties of the deposited component, finite element modeling can be utilized. However, because of several restrictions, there are still gaps between current LAAM models and mimicking real-world industrial applications.
One of them is limited scalability, which refers to an increase in the processing time for deposition. This restriction is partly brought about by the complexity of the laser-aided additive manufacturing process, as it often takes days or even weeks of simulation to provide findings that are sufficiently accurate.
Another drawback is that the creation of prediction models for the multi-layer deposition process requires knowledge of both the LAAM experimental procedure and finite element modeling. Therefore, to effectively provide accurate prediction for 3D printing or wide area surface deposition processes, more improvement in numerical models and other unique prediction approaches is required.
The machine learning method entails creating and using computer algorithms to create predictions, which become more accurate as input datasets get bigger. It has been used in a variety of contexts, including strategy planning, optimization, and calls for field integration.
However, very few studies have documented the use of machine learning algorithms in manufacturing, particularly in additive manufacturing (AM). This may be because of two factors: first, it takes a lot of resources to collect sufficient amounts of large-scale data for training, and second, labeling data typically necessitates connecting the value of the data with its physical meaning, which necessitates laborious data preprocessing like classifying and filtering. Because training data for AM are relatively easy to get, early research efforts concentrated on process monitoring with image processing, and flaws identification.
Machine learning algorithms may benefit from training data from finite element models. The next challenge is to produce enough precise data for the chosen study parameter. The complexity of the LAAM finite element model, which often comprises several process parameters linked to laser beam, material powder, and substrate, makes it more challenging to create a dataset for the machine learning technique.
Documenting and presenting such detailed simulations in research papers or technical reports is equally demanding. For researchers who require professional assistance to accurately and effectively present their findings, services like WritePaper can be invaluable. They sponsored this article and their services ensure that complex technical content is conveyed in a clear, concise, and accurate manner, which is essential for academic publishing and industry reporting.
It is also challenging to choose an acceptable data structure to explain how the deposition state changes throughout the process. The researchers in China and Singapore have discussed and presented the possibility and approach of using machine learning algorithms with input from LAAM numerical models to evaluate laser scanning patterns.
According to their research, a combined Recurrent Neural Networks and Deep Neural Network (RNN-DNN) model for LAAM thermal analysis is suggested for the aforementioned aim. They take the following procedures to establish the model.
First, during the whole LAAM process, novel finite element thermal analysis simulation architecture is used to constantly anticipate the temperature field over the entire simulation domain. The huge thermal history dataset is produced using the developed finite element model as a base. Second, to characterize the laser scanning state and associated temperature field during the deposition process, a special dataset structure is created. Thirdly, the RNN-DNN model’s structure is created to predict temperature behavior based on a specific laser scanning status. In the rest of this article, we briefly introduce this novel methodology.
Thermal analyses for laser-aided additive manufacturing
In laser aided additive manufacturing, material powders are supplied to the substrate by a discrete coaxial powder nozzle utilizing carrier gas, where they are then melted by a powerful laser beam. For laser movement to deposit powder that solidifies to produce clad tracks, the nozzle and laser optics system are installed on a robotic arm.
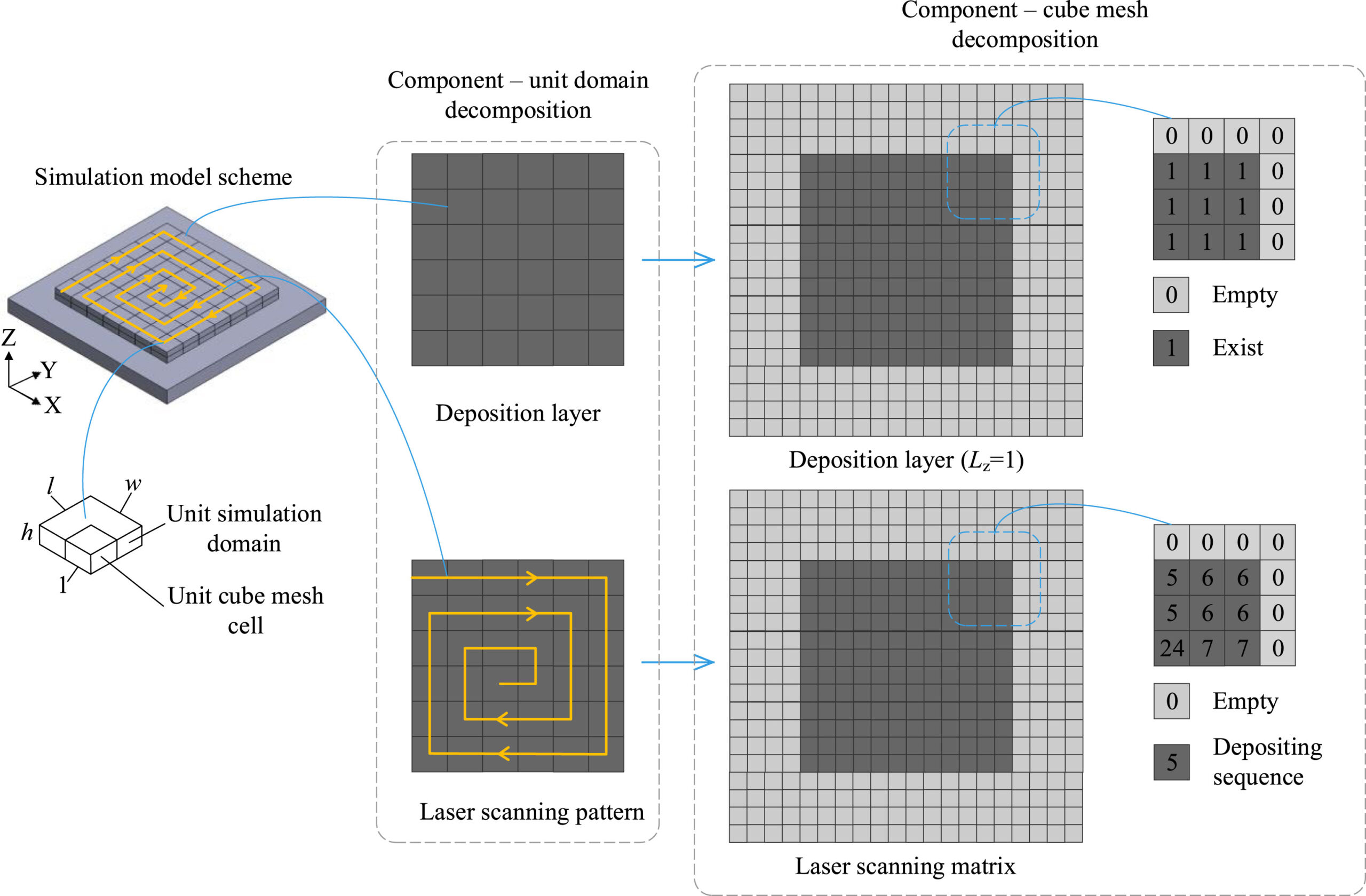
Figure 2: Component decomposition with unit simulation domain and unit mesh cell. Photo courtesy of Elsevier
When a unit simulation phase is finished, the temperature field is saved and imported as the starting condition for the simulation of the following step. A predetermined laser scanning pattern is used to merge various unit simulation domains. The simulation for the entire deposition process is made up of the propagation of all unit simulation steps. Figure 1 depicts the development of the modeling architecture generation.
Results
Throughout the whole laser-aided additive manufacturing process, the temperature field evolution is continually predicted using a cutting-edge thermal analysis finite element model. Based on a created data structure, the discretized deposition status and associated temperature field of the entire simulation domain are retrieved from the finite element model. Then, using 100 distinct one-layer deposition scenarios with six unique laser scanning procedures each, a LAAM thermal history dataset is created, having a total of over 47,000 deposition states. Figure 2 and Figure 3 show the schematic of this procedure.
When compared to the finite element model, the prediction accuracy of the relationship between various laser scanning patterns and the output temperature field is trained using an RNN-DNN model. The created RNN-DNN model can swiftly compare various laser scanning patterns and can greatly increase prediction accuracy, as we can see in Figure 4. The created structure might be expanded for future study in order to do thermal research of a multi-layered 3D deposition process.
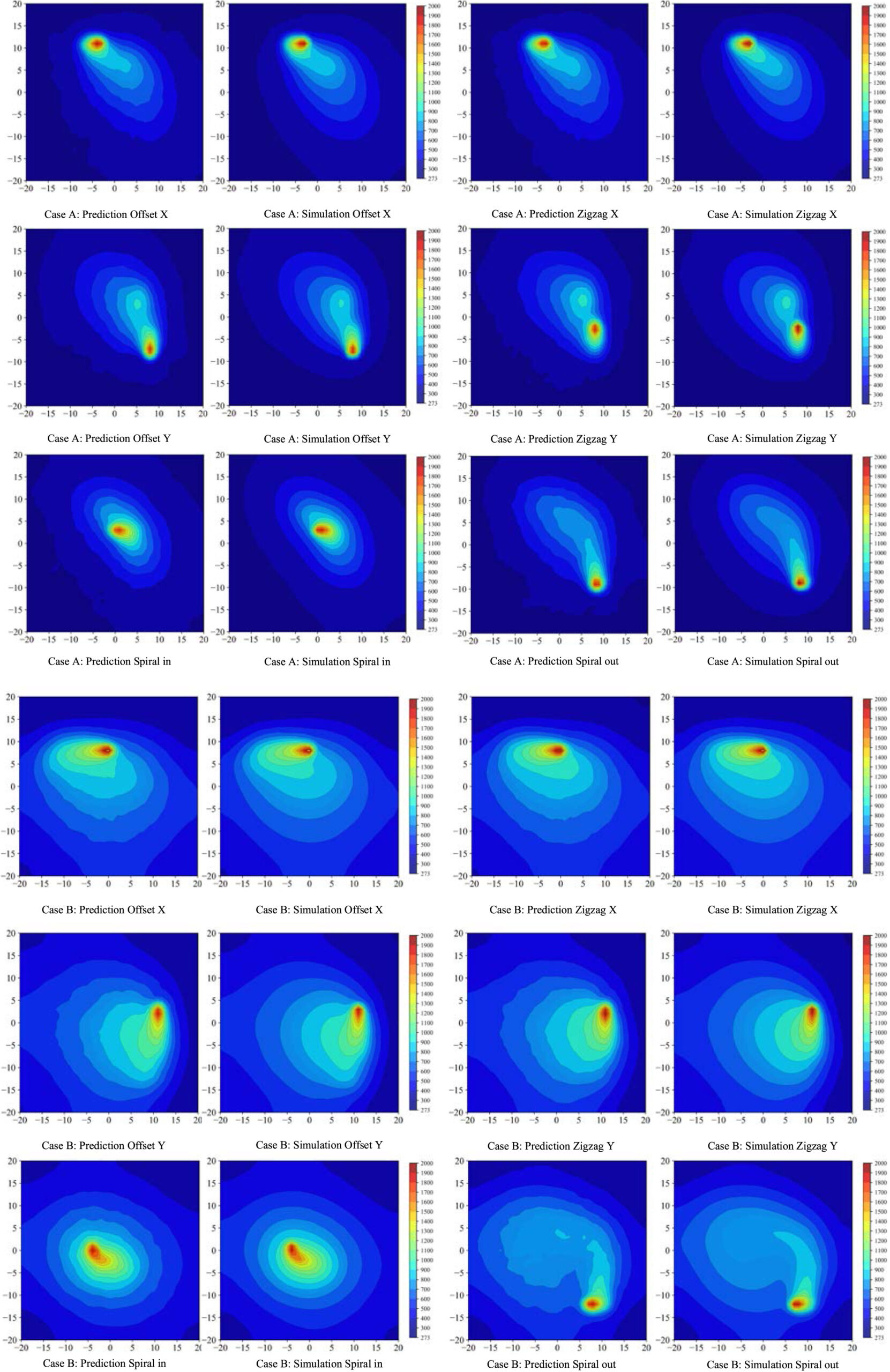
Figure 4: Comparison between machine learning prediction and finite element model simulation for the temperature. Photo courtesy of Elsevier