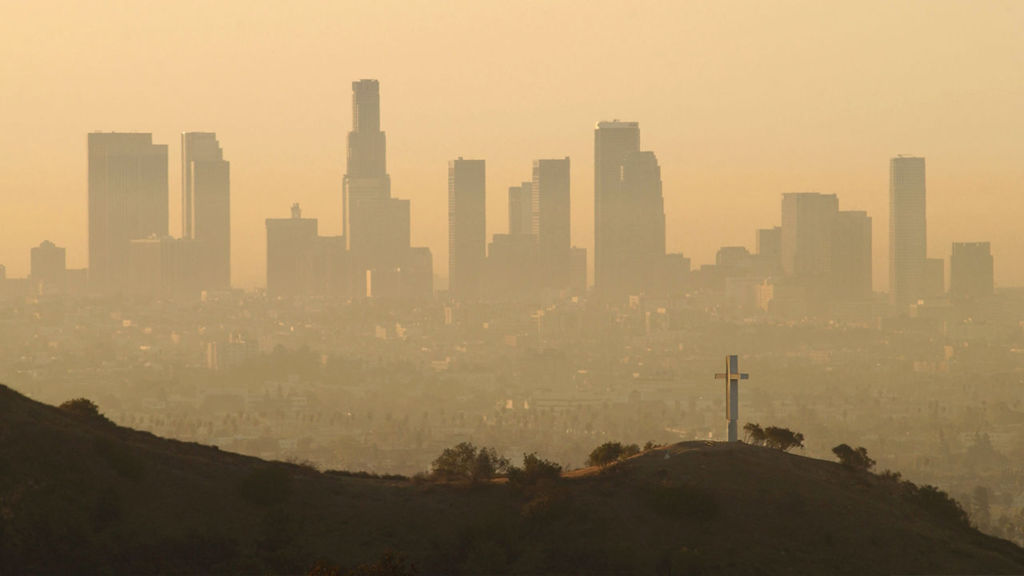
Neural networks could play a crucial role in characterizing particles that pollute the air and affect human health. Courtesy of Science Magazine.
The Impact of Particle Pollution
Environmental pollution is directly linked to a long list of health problems including asthma, decreased lung function, and cardiovascular disease. A study conducted by a collaborative team of researchers from around the world found that outdoor air pollution leads to 3.3 million premature deaths per year worldwide. This global health crisis requires in-depth surveillance of particulate matter.
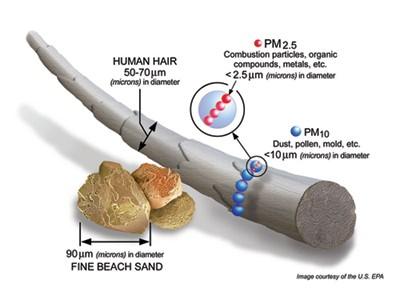
The above graphic shows both the size of coarse (PM10) and fine (PM2.5) particles in comparison to a human hair. Courtesy of EPA
What is Particle Pollution?
Particulate matter, also known as particle pollution, refers to a combination of very small solid and liquid particles in the air. This encompasses a wide variety of components from metals to molds. Some of these particles like dust, soot, and dirt are visible to the naked eye; others can only be viewed with sophisticated electron microscopy equipment. Particles that are 2.5 micrometers wide or smaller are classified as “fine particles.” Smaller particles pose the biggest threat as they pass through the nose and throat to enter the lungs.
As we learn more about the health risks, researchers have developed techniques to characterize particles that contribute to particle pollution. Gaining qualitative and quantitative information about samples from the air facilitates a system of environment monitoring that could directly impact human health.
Particle Pollution Sensing and Characterization
Scattered light is the key to characterization. Light scattered from an object carries with it a wealth of information about the structure and makeup of that object. Decrypting all of this information, however, requires specialized equipment. Researchers have developed approaches such as phase retrieval and ptychography in order to gain access to the complete scattering pattern of an object. While these methods of mapping are effective, they place tight constraints on the sample size and illumination function.
A research team at University of Southampton published their study of particle pollutant sensing in an October issue of Optics Express. The team believes neural-networks could be the key to lensless imaging. Neural networks are a computing system inspired by the network of neurons in biological species. It is a framework for machine learning- that is, a method of processing complex dating without programming. Here, the network classifies objects through scattering media and diffraction imaging. Using neural networks allows the researchers to characterize particles without the device “understanding” light-matter interaction. Their work demonstrates the ability to determine size, material, and particle count using machine learning.
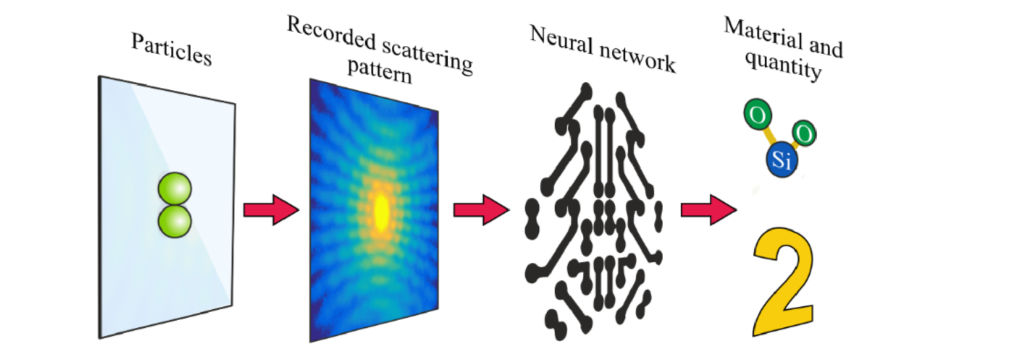
The above image shows the process of neural networks quantifying scatter patterns. Courtesy of OSA Publishing.
The Experiment
The initial setup is typical of many scattering experiments: a HeNe laser focuses on a substrate causing light to scatter onto a screen where it is imaged by a CMOS camera. To train the network, the team first inserts a material with known particle distribution and particle count. The network then generates a training data set consisting of inputs (single scattering patterns) and outputs of interest (materials and number of particles). The set consists of 568 items.
This experiment addresses two aspects of particle pollution monitoring: particulate mapping and real-time sensing. The research team chose to run their mapping tests on plastic microspheres, a major source of marine particle pollution. They acquired hundreds of scattering patterns from samples of different makeup to predict particle count and position. The team then determined their method’s ability to sense airborne pollution in real-time. Here they tested their approach on airborne pollution including two types of pollen grains, diesel soot, and wood ash. After training, the neural network took less than 50 milliseconds to record and process each scattering pattern.
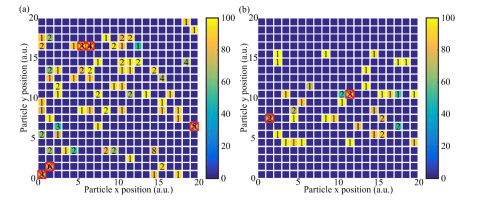
Particle maps for (a) polystyrene and (b) silicon dioxide spheres. The maps show the predicted material and number of particles at each position. Courtesy of OSA Publishing.
The Results
Both of these applications produced mixed results. The accuracy of the predicted maps seem to vary with particle count. Scattering patterns with zero particles had a 100% accuracy while three or more particles yielded only a 40% accuracy. The team attributes this decline to the relative rarity of such scattering patterns in the training set. For the real-time measurements, the neural network seemed to struggle to accurately identify certain pollutant types. While the system correctly identified Narcissus pollen grains 86% of the time, it was only able to identify diesel soot with 40% accuracy. The research team writes that this discrepancy is likely due to ease of identification of features in the scattering patterns.
Looking Forward
The technology is still young, and the neural networks require more training to more accurately place and identify certain particles. This real-time method, however, shows great promise. Particle pollution poses a serious threat to the health of millions worldwide. Detection and identification of these particles is the first and most important step in addressing environmental pollution. With some fine-tuning, machine learning could make a huge impact on modern particle pollution detection.
For more information about their critical research, find the University of Southampton team’s paper here.